LTP ROBUST
Robust Trustworthy Al-based Systems for Sustainable Growth
Overview
The AI for Energy Grids Lab extends the Innovation Center for AI and is part of the Long Term Program on Trustworthy Al-based Systems for Sustainable Growth. The Lab will develop algorithms for energy grids and focus on two main ways of extending grid capabilities, one focusing on improving grid operations and the other on targeted infrastructure expansions. In particular, the Lab will analyse large-scale, noisy grid data and develop reliable and safe AI-based methods that learn from this type of data to support the extension of grid capabilities. Two PhD projects within IEPG will work on state estimation and risk-based investment and operation.
Project description
The project on state estimation is motivated by the given the new dynamics brought to the system by distributed renewable energy resources and cutting-edge technologies; DSOs must develop a more active role in monitoring the network. For this purpose, state estimation may become a fundamental task for DSOs. However, implementing state estimation for distribution networks presents many challenges, not only because distribution grids need more observability but because the network size makes conventional algorithms inaccurate or fast enough. Many developments in machine learning applied to power systems are being researched to achieve what conventional algorithms do not accomplish. Considering that state estimators of the future must be more flexible to account for future tasks, such as anomaly detection, congestion management, fraud detection or flexibility provision, researching how to incorporate ML into the state estimation task seems like a promising direction.
The methodologies being researched in this project includes:
• GNNs and Bayesian methods
• physics-constrained learning with grid topology
• task-based learning
• transfer learning
• advanced neural network architectures
• real-time one-shot learning
This project is working closely with Alliander's open-source Power Grid model and their IT systems, where real data of distribution networks is being provided.
The second project, risk-based investment and operation is to identify grid congestion risks and to rank appropriate mitigation options. Mitigation options range from traditional network to market-based flexibility mechanisms. Achieving this goal requires developing a decision support methodology that addresses three sub-goals:
(1) Integrate network models and measurements to learn spatiotemporal models of network usage and available flexibility options, including network switching, which influences forecasts.
(2) Generate probabilistic forecasts of remaining capacity that are calibrated on the tails (low remaining capacity) and quantifies uncertainties due to lack of input data.
(3) Provides a risk-based ranking of mitigation options, including time-to-decide, and scales to online use for large networks with thousands of potentially congested assets.
Intended deliverables
• Design a real-time state estimator for distribution networks
• Impact analysis of the state estimator and comparison to standard algorithms
• Design of an automated, calibrated and risk-aware method for quantification of congestion risks and comparison of mitigation options.
• Implementation of methodology in in-house and open-source forecasting and planning tools used by Alliander and other DSOs.
Project partners
Alliander N.V., Radboud University, University of Twente, HAN University of Applied Sciences, AI for Energy Grids Lab
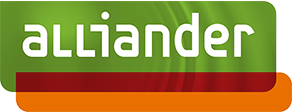
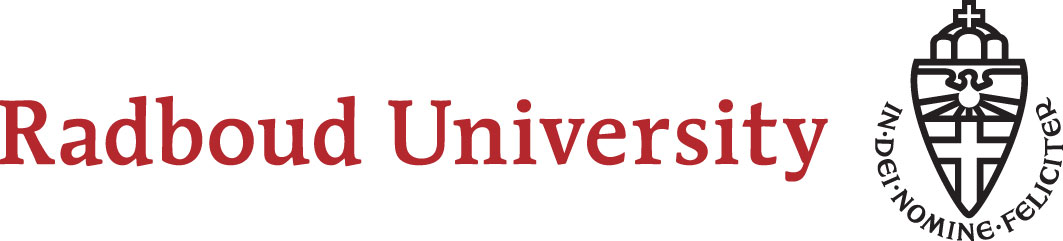
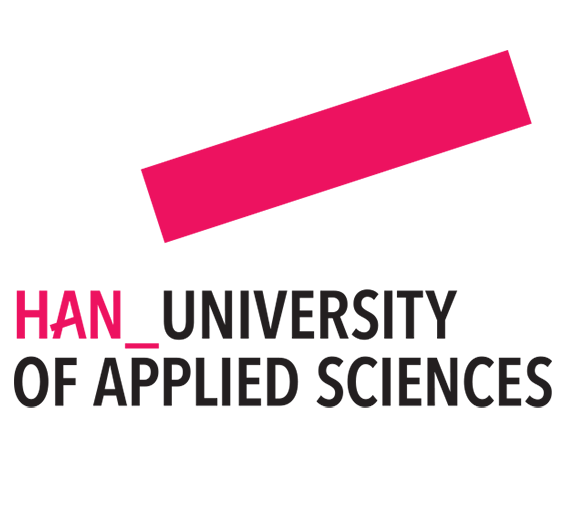
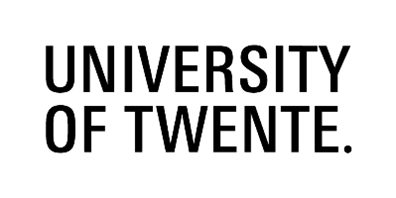
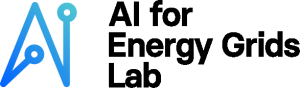
TUD team
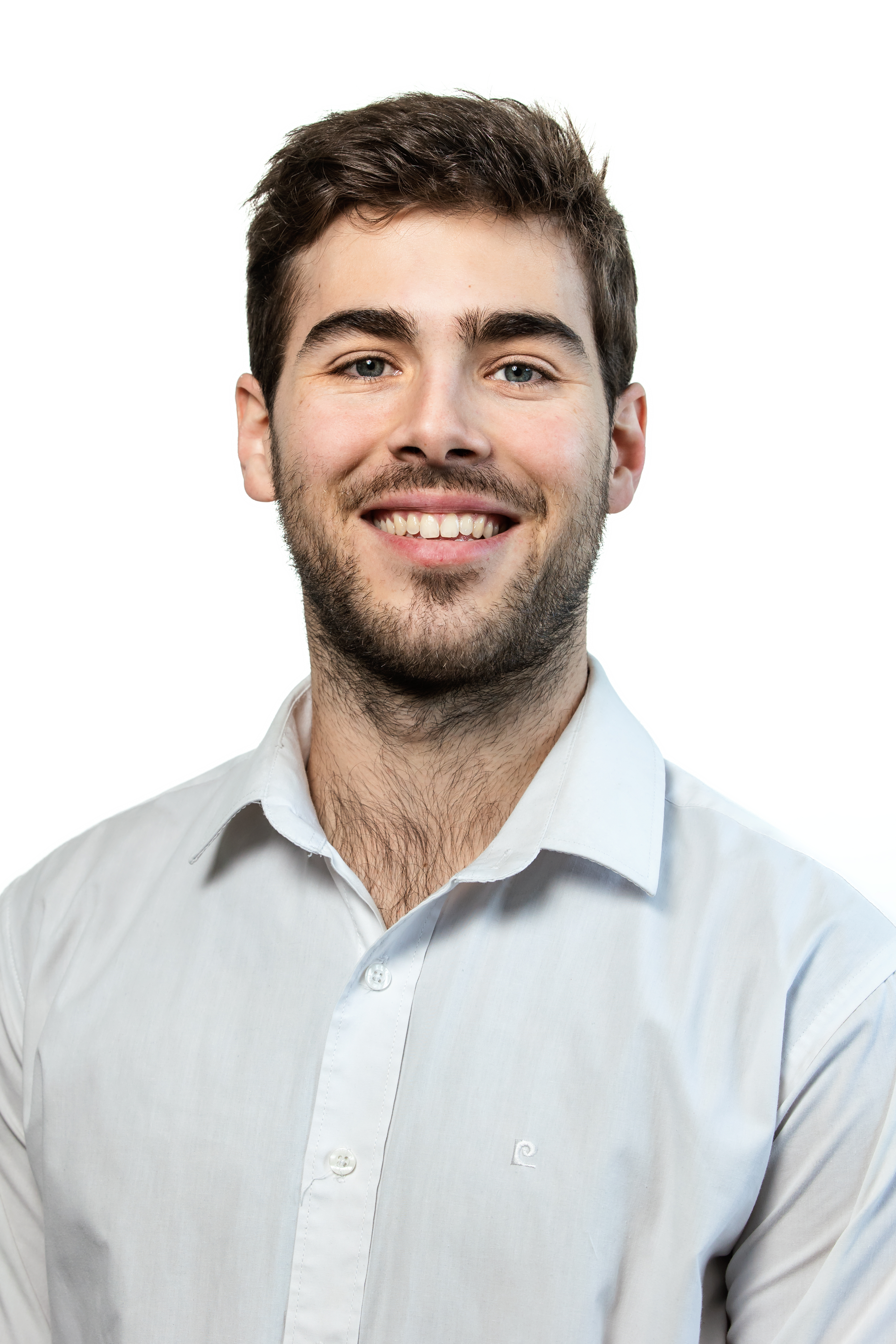
PhD Researcher:
L. (Lucas) Narbondo
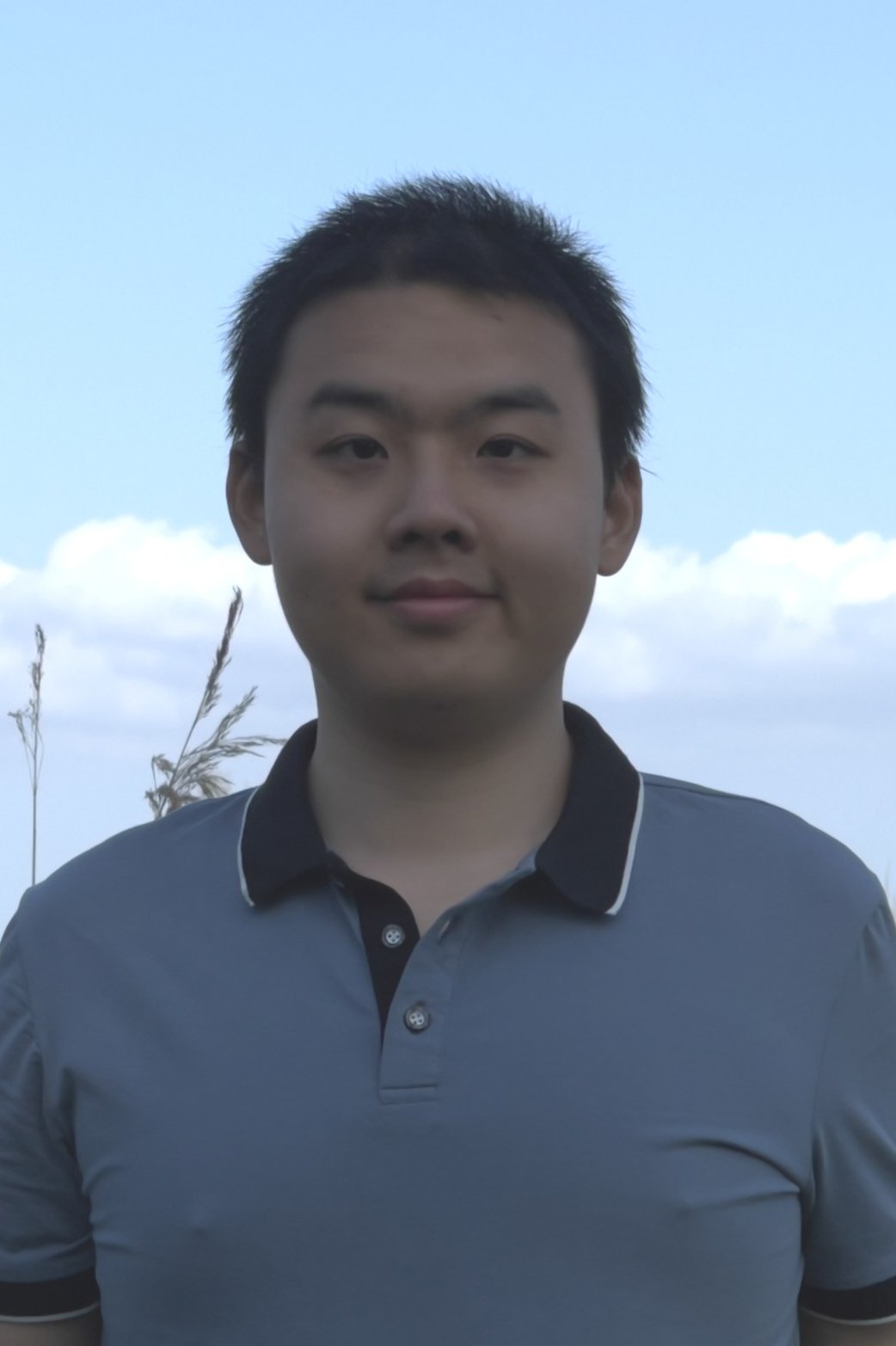
PhD Researcher:
S. (Shaohong) Shi
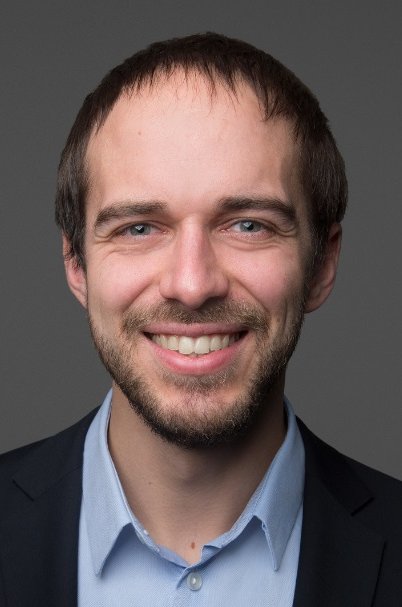
Responsible:
Dr. J. (Jochen) Cremer
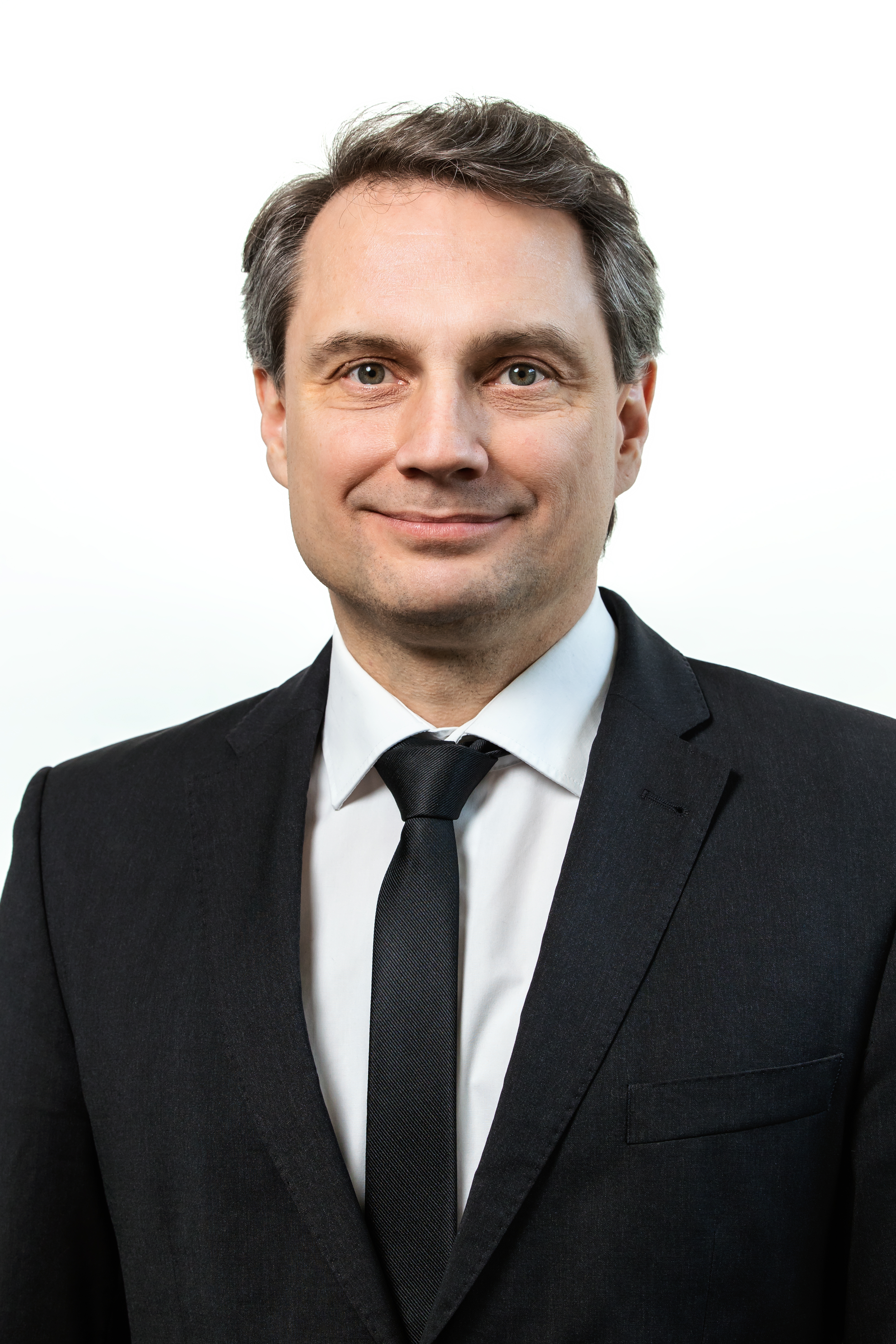
Responsible:
Prof.dr. P. (Peter) Palensky
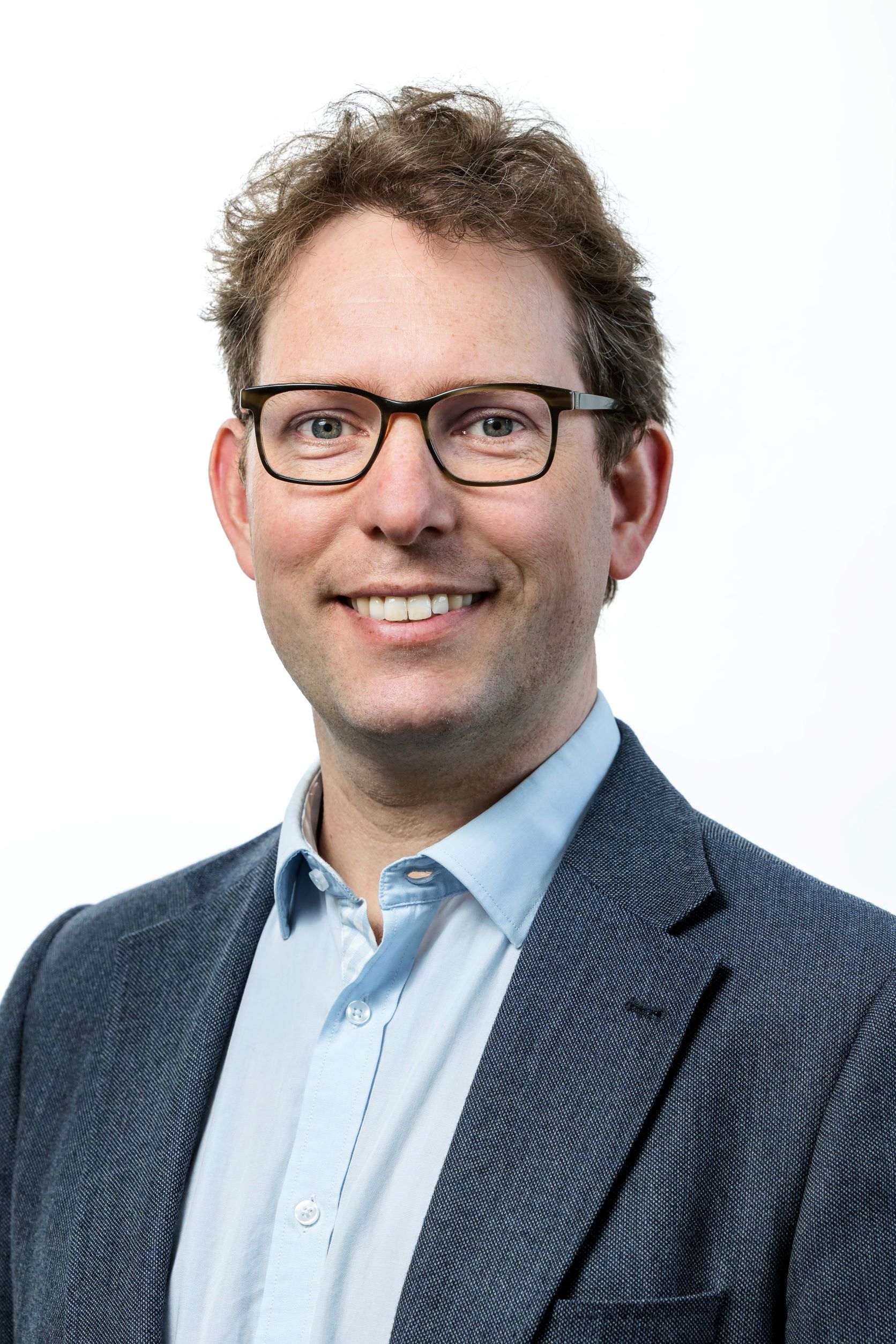
Responsible:
Dr. S. (Simon) Tindemans
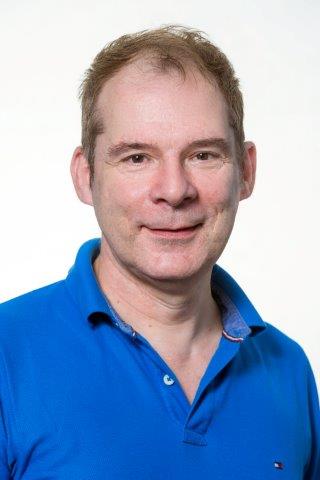
Responsible:
Prof.dr.ir. Han La Poutre
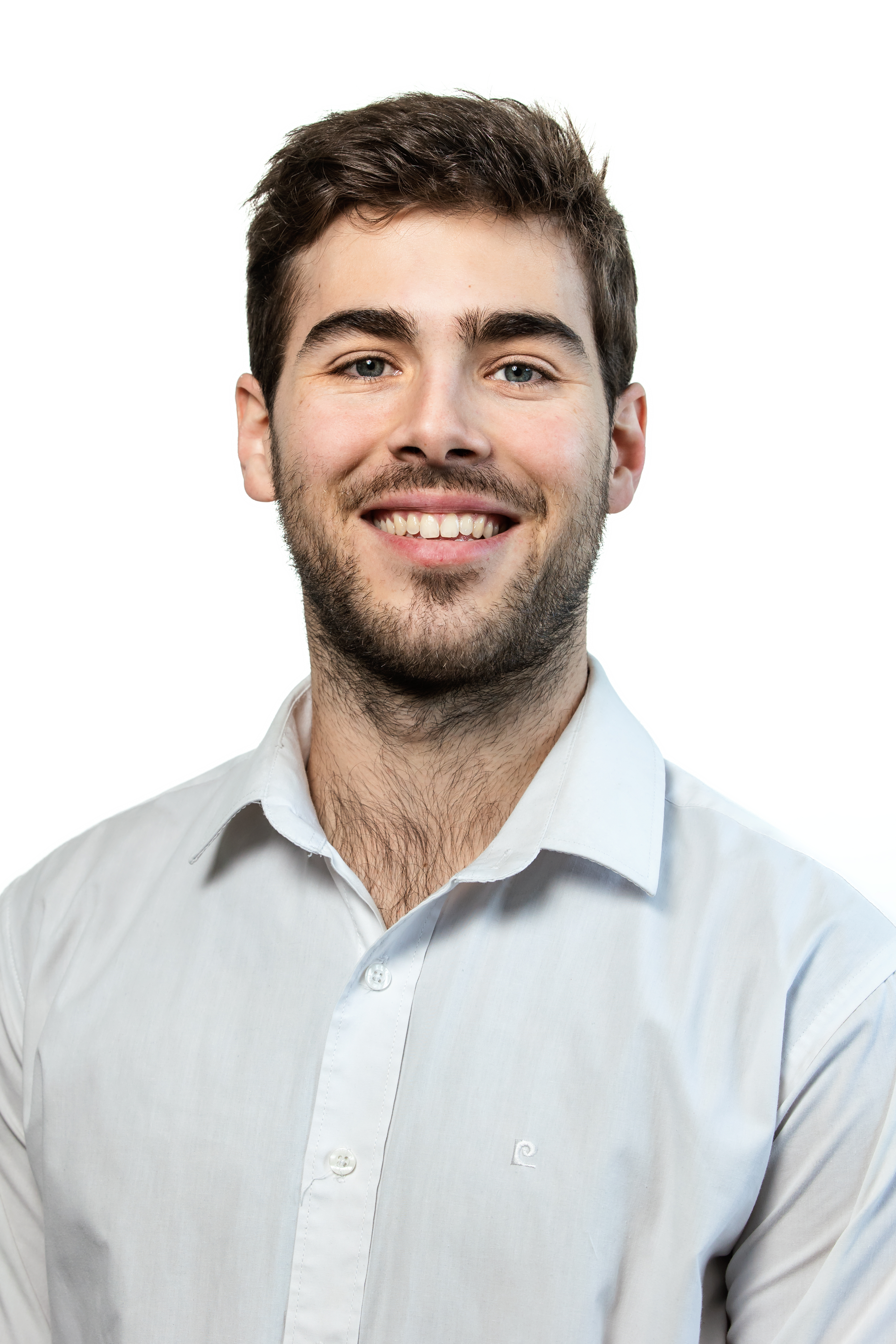
L. (Lucas) Narbondo
Lucas joined TU Delft in November 2022 as a PhD Candidate at Delft AI Energy Lab. He is working in collaboration with Alliander to study how to incorporate machine learning techniques to improve the state estimation of distribution networks. He is also a member of the IEPG group and the EEMCS department. Lucas obtained his electrical engineering degree at Universidad de la Republica, Uruguay, and his master's in Future Power Networks at Imperial College London under the Chevening scholarship. He has vast experience in the renewable energy industry, which includes academia and the private and public sectors.
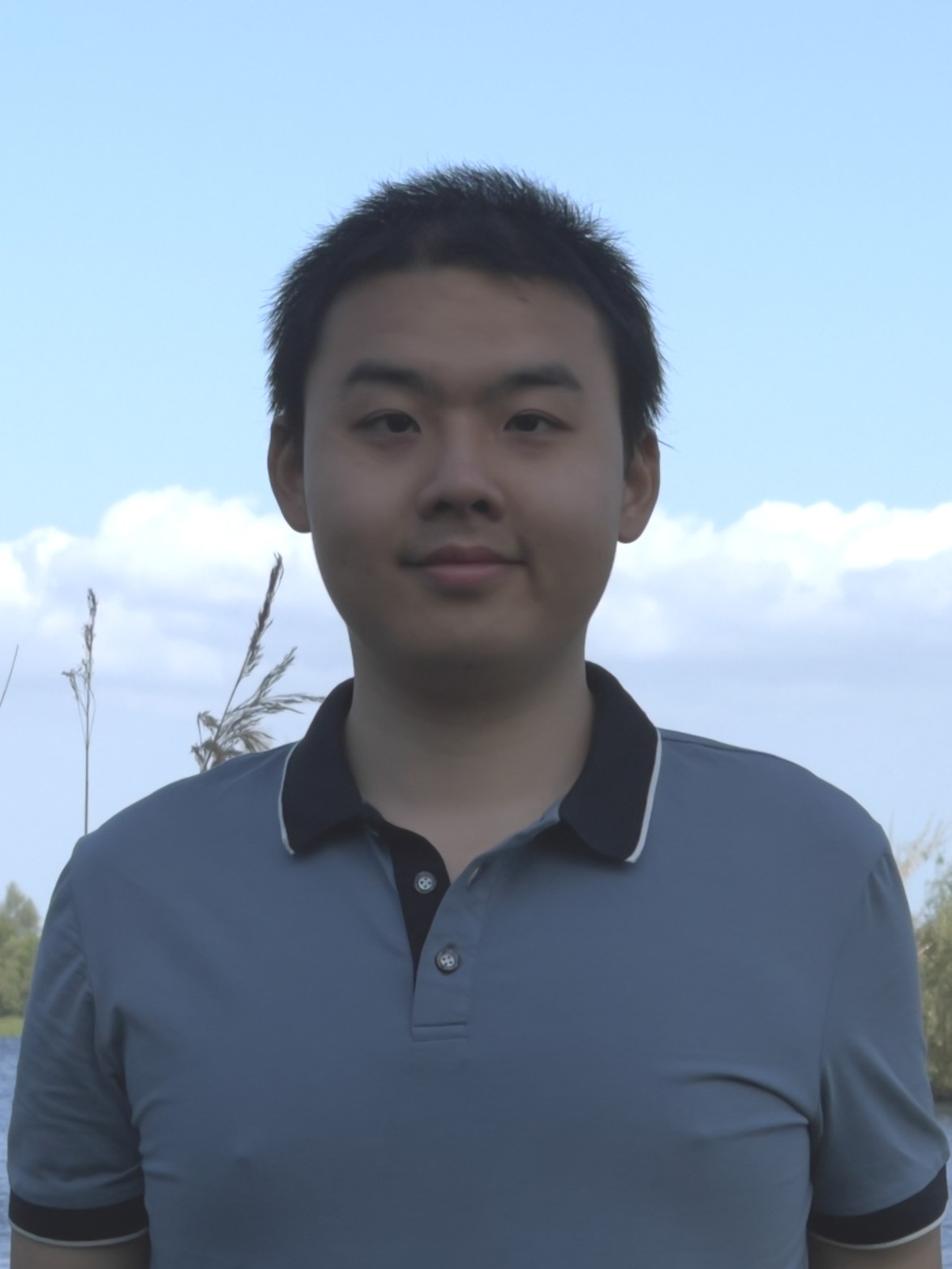
S. (Shaohong) Shi
Shaohong joined TU Delft in September 2023 as a PhD candidate in the IPEG group of the EEMCS department. He received his Bachelor’s degree in Mathematics and Applied Mathematics from Peking University, China in 2020. He received his Master’s degree in Mathematics from Leiden University, the Netherlands in 2023. His area of interest is the combination of statistical model and machine learning and its applications to probabilistic forecasting in power systems. His doctoral research is focused on congestion headroom and flexibility options for medium-term planning.