European Summer School in Financial Mathematics
04 September 2023 09:00 till 08 September 2023 17:00 | Add to my calendar
The European Summer School in Financial Mathematics was initiated in Paris in 2009 and has been hosted by various European cities throughout the years. This year it will be hosted in the Netherlands for the first time. The leading institution in the organization will be TU Delft, while U Amsterdam, U Utrecht and TU Eindhoven will also participate in the organization.
The 15th European Summer School in Financial Mathematics will be hosted by the Delft Institute of Applied Mathematics, TU Delft. The main theme of the summer school will be
Mathematics of FinTech
and the following topics will be addressed:
- Hansjörg Albrecher: Blockchains and Decentralized Insurance
- Martin Kroll: Introduction to Differential Privacy
- Kees Oosterlee: Machine Learning for Anti-Money Laundering and Deep Portfolio Optimisation in Finance
- Jörg Osterrieder: Artificial Intelligence and Deep Reinforcement Learning in Finance
In addition to the main courses, there will be talks from PhD students and early career researchers, as well as an afternoon of presentations by practitioners.
The European Summer School in Financial Mathematics aims at bringing together the most talented young researchers in mathematical finance. PhD students and early career researchers are invited to submit their application for participation at the following link by 4 June 2023. Partial financial support is also available.
The presentations by PhD students and early career researchers, as well as the discussion sessions will allow participants to engage with each other and discuss their current research. One of the aims of these summer schools is to encourage active cooperation and collaboration in mathematical finance among European institutions.
This school belongs to the series of the EMS Applied Mathematics schools. We gratefully acknowledge the generous contribution of various sponsors for financial support.
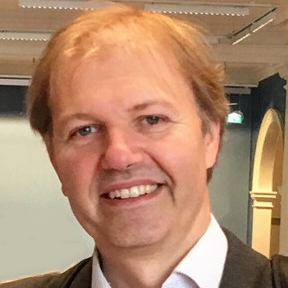
Hansjörg Albrecher
University of Lausanne
Blockchains and Decentralized Insurance
In this minicourse, we give an overview of the concept of blockchains, which are distributed data ledgers based on consensus among different nodes in a peer-to-peer network. We discuss various consensus protocols and evaluation criteria such as efficiency, decentralization and security, and we review mathematical models and tools to assess the performance of blockchain systems. For a resource-consuming proof-of-work based blockchain (as is for instance used for the crypto-currency Bitcoin) we then study the trade-off between profitability and risk for miners in more detail, adapting tools from insurance risk theory, which will also allow to quantify the efficiency of mining pools and selfish mining strategies. Finally, we give an overview of recent developments and the potential of decentralized insurance.
Hansjörg Albrecher is Professor of Actuarial Science at the Faculty of Business and Economics, University of Lausanne and a Faculty Member of the Swiss Finance Institute. After studying in Graz, Limerick and Baltimore, he held faculty and visiting positions in Graz, Leuven, Aarhus and the Radon Institute of the Austrian Academy of Sciences in Linz before moving to Lausanne in 2009, and guest professorships at various institutions since then, including the University of Hong Kong and ETH Zurich. His research interests lie in all kinds of risk modelling in insurance and finance, particularly using tools from analysis and applied probability.

Martin Kroll
University of Bayreuth
Introduction to Differential Privacy
This mini-course is supposed to provide an introduction to differential privacy. It is divided into four parts. In the first part, we motivate the need for privacy-preserving data analysis. We discuss several notions of privacy circulating in the research literature and discuss the definition and basic properties of differential privacy and its nearby relatives in detail. The second part is mainly algorithmic. We introduce the main methods used to generate differentially private data. The third and the fourth part are then devoted to the analysis of differentially private data. In the third part we discuss the case of global differential privacy, whereas the fourth and final part concerns the more restrictive notion of local differential privacy. In this last part I will also present some results related to my own research.
Martin Kroll obtained a PhD in Mathematics from the University of Mannheim in 2017. He was a postdoctoral researcher at the University of Mannheim, École Nationale de la Statistique et de l'Administration Économique (ENSAE) and Ruhr-Universität Bochum. He is currently working as a senior research associate at the University of Bayreuth. His research interests lie in the area of nonparametric statistics.
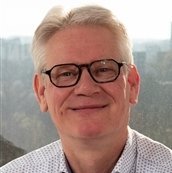
Kees Oosterlee
Utrecht University
Machine Learning for Anti-Money Laundering and Deep Portfolio Optimisation in Finance
This 4.5 hours lecture series deals with two separate topics from the financial industry, Anti-Money Laundering and Deep Portfolio Optimisation in Finance. For both topics, in modern machine learning techniques have become imperative, due to the amount of data being processes and due to the problem dimensionality in practice.
Anti-money laundering is a very important topic for the financial industry and falls under the category of fraud detection. Essentially, one searches for outliers in high-dimensional data sets. This part of the day, we start with a light historical overview of the origin of money and the history fraud in finance, after which we dive into anomaly detection to combat fraud in finance. After a brief introduction to rule-based (supervised) anomaly detection, we'll explain the details of unsupervised machine learning. Many unsupervised anomaly detection algorithms rely on the concept of nearest neighbours to compute the anomaly scores, like k-nearest neighbours. Isolation Forest (IF) is a relatively new branch on the tree of anomaly detection algorithms to search for outliers in data sets by means of the concept of isolation. We give an introduction into IF, its advantages and disadvantages as compared to nearest neighbour algorithms. Subsequently, we present the new and parameter-free Analytic Isolation and Distance-based Anomaly (AIDA) detection algorithm, that combines the metrics of distance with isolation. Based on AIDA, we also present the Tempered Isolation-based eXplanation (TIX) algorithm, which identifies the most relevant features characterizing an outlier, even in large multi-dimensional datasets, improving the overall explainability of the detection mechanism.
The second topic of the day is optimal portfolio asset allocation. In this part, we take the perspective of a trader, who is allowed to trade in a risk-free bond, in stocks, and also in options. The trader is allowed to trade at a set of trading dates. In order to evaluate how satisfied the investor is with the return, an objective function is used. A high quality objective function is able to numerically represent the investor's preferences of how much risk is acceptable for a certain level of potential profit. Moreover, we introduce market frictions aspects of incomplete markets and trading constraints. Regarding the market, we add transaction costs as well as a non-bankruptcy constraint and for the trading strategies, we introduce leverage constraints.
The resulting optimization problem admits to closed form strategies only in rare cases and we therefore have to rely on numerical approximations. When it is required, we use a time discretization scheme for the assets and we use empirical distributions as approximations in a Monte–Carlo fashion. The discrete counterpart (in time and/or in probability space) is then approximated by letting deep neural networks represent the trading strategies and optimizing with a gradient decent type algorithm. The neural networks that we use in our implementations are introduced in quite some detail in this presentation.
1. Anomaly detection and fraud (2.25 hours)
1.1. Welcome and overview of the day
1.2. History of money and fraud, Rule-based methods
1.3 Unsupervised learning in fraud detection
1.4. The AIDA algorithm and the TIX explanation method
2. Neural networks for optimal asset allocation in financial portfolios (2.25 hours)
2.1. Optimal asset allocation, objective functions
2.2. Classical approach with Monte Carlo simulation
2.3. Deep neural networks for optimal asset allocation in the general case.
2.4 Numerical example
Prof.dr.ir Cornelis ("Kees") Oosterlee, Chair of financial Mathematics, Mathematical Institute, Utrecht University.
Prof. Oosterlee has been working on computational problems in financial mathematics since 2000. He is co-author of two textbooks ("Multigrid" 2001, and "Mathematical Modeling and Computation in Finance", 2019), and many scientific publications. Financial derivative pricing and risk management methods he co-developed include the COS method on Fourier cosine expansions, SWIFT (Shannon Wavelet Inverse Fourier Transform method), SGBM (Stochastic Grid Bundling Method), SCMC (Stochastic Collocation Monte Carlo Method), and the Seven-League scheme (7L). Machine learning in Finance is another research interest in Oosterlee's group, where he works on optimal portfolio selection, time series and anomaly detection methods. Oosterlee has led two EU projects on risk management in finance and insurance, in collaboration with the industry, and he also worked on several Dutch national projects. He has been a guest lecturer at Oxford Uni, UK, Hitotsubashi Uni., Japan, Uni. A Coruna Spain, amongst others.
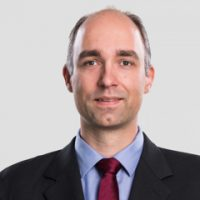
Jörg Osterrieder
University of Twente
Bern HF
Artificial Intelligence and Deep Reinforcement Learning in Finance
This lecture offers a comprehensive introduction to Artificial Intelligence (AI) and Deep Reinforcement Learning (DRL) applications in the finance industry. The course covers fundamental AI techniques, their financial applications, and the unique challenges faced in the industry. Participants will gain insights into various AI-driven solutions such as algorithmic trading, credit scoring, fraud detection, and portfolio optimization. The course also delves into the rapidly evolving field of DRL, exploring key algorithms, their underlying principles, and applications in finance, such as algorithmic trading, risk management, and option pricing.
A hands-on workshop will provide participants with practical experience in implementing a DRL model for algorithmic trading. The workshop covers essential steps, including data preprocessing, feature engineering, DRL model implementation, and performance evaluation. Participants will have the opportunity to work with real-world financial data and apply their learnings to develop a trading strategy using DRL.
This lecture is designed for PhD students and early-career researchers interested in the intersection of AI, DRL, and finance. By the end of the course, participants will have a solid understanding of AI applications in finance, DRL techniques, and the practical know-how to implement and evaluate DRL-driven financial strategies.
1. Introduction
1.1. Welcome and overview
1.2. Basics of finance and financial markets
1.3. Introduction to Artificial Intelligence (AI) and Machine Learning (ML)
1.4. Role of AI and ML in finance
2. Artificial Intelligence in Finance
2.1. Overview of AI techniques in finance 2.1.1. Supervised learning 2.1.2. Unsupervised learning 2.1.3. Reinforcement learning
2.2. AI applications in finance 2.2.1. Algorithmic trading 2.2.2. Credit scoring and risk assessment 2.2.3. Fraud detection 2.2.4. Portfolio optimization 2.2.5. Financial forecasting 2.2.6. Chatbots and customer service
2.3. Key considerations and challenges 2.3.1. Data quality and availability 2.3.2. Model interpretability and explainability 2.3.3. Ethical and regulatory issues
3. Deep Reinforcement Learning in Finance
3.1. Introduction to Reinforcement Learning (RL) 3.1.1. RL framework: agents, environments, and interactions 3.1.2. Markov Decision Processes (MDPs) 3.1.3. Exploration vs. exploitation 3.1.4. Policy and value functions
3.2. Deep Reinforcement Learning (DRL) algorithms 3.2.1. Value-based methods: Q-learning, Deep Q-Networks (DQN) 3.2.2. Policy-based methods: REINFORCE, Proximal Policy Optimization (PPO) 3.2.3. Actor-critic methods: Advantage Actor-Critic (A2C), Deep Deterministic Policy Gradients (DDPG)
3.3. DRL applications in finance 3.3.1. Algorithmic trading and market making 3.3.2. Portfolio optimization and asset allocation 3.3.3. Risk management 3.3.4. Option pricing and hedging
3.4. Practical aspects of DRL in finance 3.4.1. Data preprocessing and feature engineering 3.4.2. Training and evaluation of DRL models 3.4.3. Hyperparameter tuning and model selection 3.4.4. Overfitting and generalization
4. Hands-on Workshop: Deep Reinforcement Learning for Algorithmic Trading
4.1. Introduction to the workshop 4.1.1. Overview of the problem and objectives 4.1.2. Dataset and tools 4.2. Preprocessing and feature engineering 4.2.1. Data cleaning and normalization 4.2.2. Feature selection and extraction 4.2.3. Data splitting: training, validation, and testing
4.3. Implementing a DRL algorithm 4.3.1. Selecting a suitable DRL algorithm 4.3.2. Defining the environment, agent, and reward function 4.3.3. Implementing the DRL model using a suitable framework (e.g., TensorFlow, PyTorch)
4.4. Training and evaluation 4.4.1. Training the DRL model 4.4.2. Evaluating the model on validation and test data 4.4.3. Assessing the performance of the trading strategy
4.5. Wrap-up and discussion 4.5.1. Sharing results and insights 4.5.2. Challenges faced and lessons learned 4.5.3. Potential improvements and future work
5. Conclusion
5.1. Recap of key takeaways
5.2. Future trends and research directions
5.3. Closing remarks and end of the session
Prof. Dr. Jörg Robert Osterrieder, Professor of Sustainable Finance, Bern Business School and Associate Professor of Finance and Artificial Intelligence, University of Twente
Joerg Osterrieder is Associate Professor of Finance and Artificial Intelligence at the University of Twente in the Netherlands, Professor of Sustainable Finance at Bern Business School in Switzerland, and Advisor on Artificial Intelligence to the ING Group's Global Data Analytics Team. He has more than 15 years of experience in financial statistics, quantitative finance, algorithmic trading, and the digitization of the finance industry.
Joerg is the Chair of the European COST Action 19130 Fintech and Artificial Intelligence in Finance, an interdisciplinary research network comprised of over 200 researchers from 49 countries.
He is the director of studies for an executive education course titled "Blockchain, Machine Learning, and Data Science in Finance" and the primary organizer of a series of annual research conferences on Artificial Intelligence in Finance. He is a founding associate editor of Digital Finance, editor of Frontiers Artificial Intelligence in Finance, and frequent reviewer for leading academic journals. He also serves as an expert reviewer for the European Commission's "Executive Agency for Small and Medium-Sized Enterprises" and "European Innovation Council Accelerator Pilot" programs.
In close collaboration with the Finance industry, he has led or co-led over thirty national and international research projects on a wide range of quantitative, data-driven topics over the past few years.
Previously he worked as an executive director at Goldman Sachs and Merrill Lynch, as quantitative analyst at AHL as well as a member of the senior management at Credit Suisse Group. Joerg is now active at the intersection of academia and industry, focusing on the implementation of research results in the financial services industry.
Schedule
Monday | Tuesday | Wednesday | Thursday | Friday | |
09.00-10.30 | Kroll | Albrecher | Osterrieder | Osterrieder | Oosterlee |
10:30-11:00 | Break | Break | Break | Break | Break |
11.00-12.30 | Kroll | Albrecher | Osterrieder | Oosterlee | Oosterlee |
12.30-14.00 | Lunch | Lunch / Poster | Lunch / Poster | Lunch / Poster | Lunch |
14.00-15.30 | Albrecher | Kroll | Osterrieder | ||
15.30-16:00 | Break | Break | Break | practitioners afternoon | |
16.00-18.00 | Students presentations | Poster sessions | Students presentations | ||
19.00-21.00 | Dinner |
The Book of Abstracts for the summer school is available here (pdf).
The summer school will take place at the following locations:
Monday:
Vakwerkhuis
Professor Snijdersstraat 2
2628 RA Delft
https://vakwerkhuis.com/
Tuesday until Friday:
X (Building 37)
Theatre Hall
Mekelweg 8
2628 CD Delft
https://map.tudelftcampus.nl/nl/poi/x/
- Flavia Barsotti
- Alexis Derumigny
- Fang Fang
- Ludolf Meester
- Kees Oosterlee (Utrecht)
- Antonis Papapantoleon
- Elisa Peronne (TU Eindhoven)
- Peter Spreij (UvA)
- Fenghui Yu
The Scientific Committee consists of European leaders and representatives of financial mathematics. We warmly thank them for their encouragement and for accepting to be part of this committee.
Peter Bank, Peter Imkeller, Wolfgang Runggaldier, Mete Soner, Youri Kabanov, Walter Schachermayer, Josef Teichmann, Santiago Carillo, Ralf Korn, Martin Schweizer, Albert Shiryaev, Nicole El Karoui, Gilles Pagès, Huyen Pham, Marco Frittelli, Damien Lamberton, Bernard Lapeyre, Lukas Stettner, David Hobson, Bernt Øksendal, Denis Talay, Chris Rogers