Finding the sweet spot for transparency and control in music recommendations
In music recommender systems, it’s important to design user control that hits the sweet spot between the perceived quality of recommendations and acceptable cognitive load, concludes TU Delft researcher Nava Tintarev. Together with colleagues from KU Leuven, and research with the Spotify API, she will present these findings at the ACM Conference on Recommender Systems in Vancouver, on Wednesday October 3rd.
Control
'Enabling users to control the recommendation process, for instance in music recommender systems, can increase user satisfaction. However, providing additional controls also increases cognitive load, and different users have different needs for control. Therefore, we have investigated the effect of two personal characteristics: musical sophistication and visual memory capacity’, says Nava Tintarev of TU Delft.
Traditional user interfaces of recommender systems present the recommendation results with limited feedback possibilities, only allowing the users to indicate how much they like a recommendation. In contrast, Interactive Recommender Systems improve user satisfaction and perceived effectiveness by providing a visualization where users can inspect the recommender process and control the system to receive better recommendations.
Personal recommendation acceptance
To better understand the interaction between different types of controls, it is necessary to take into account the influence of an individual user’s personal characteristics and test combinations of control components. ‘Nobody has yet investigated how interaction among different control components influences the cognitive load and recommendation acceptance, for users with different personal characteristics. Our study, carried out together with KU Leuven, aims to provide the groundwork for developing recommender systems which offer rich user control, while ensuring acceptable cognitive load.’
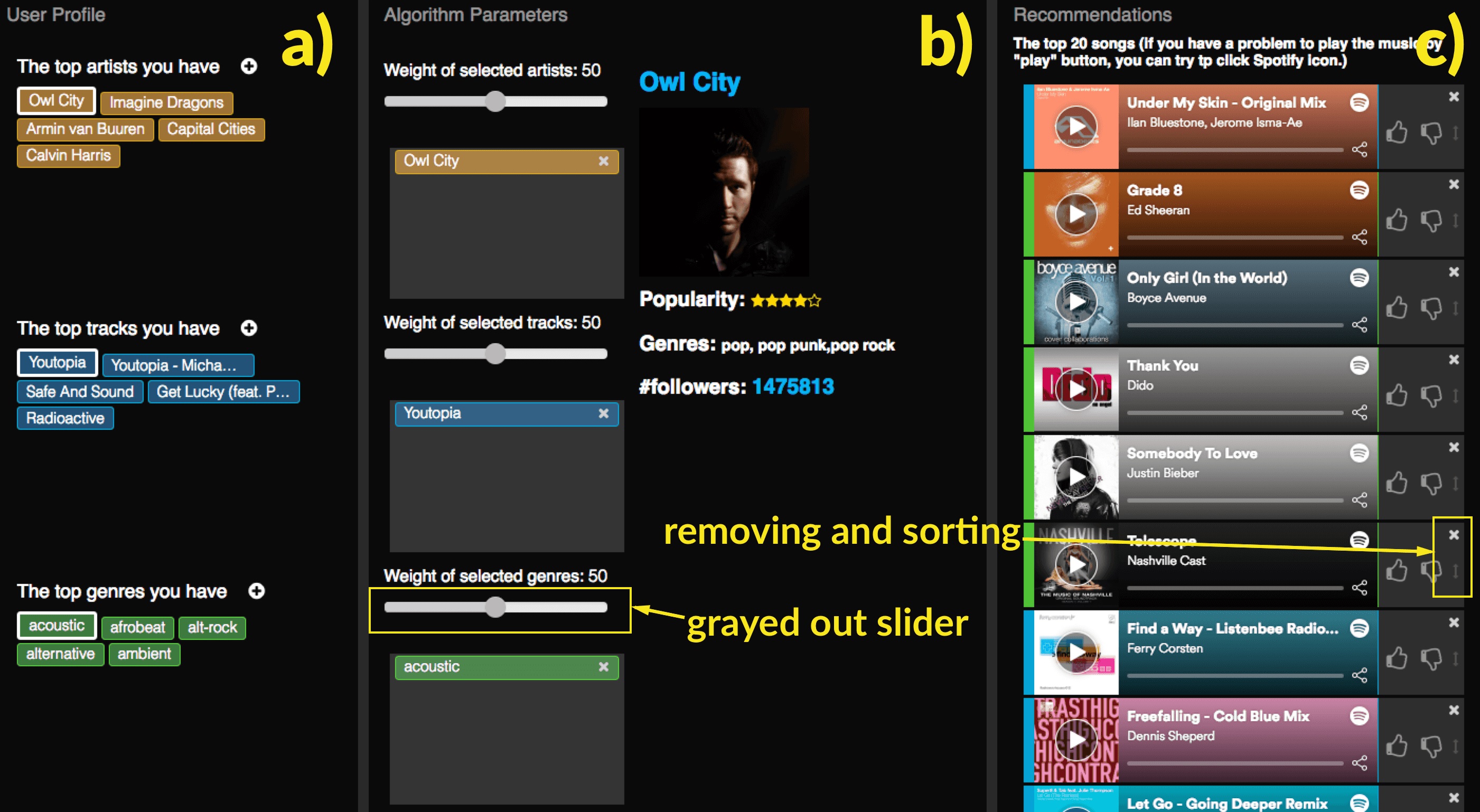
‘Rock night - my life needs passion’
‘We used the Spotify API to design a music recommender system. Our system generates a play-list style listening experience based on three types of seeds: artists, tracks, and genres. We use the active user’s top artists, tracks, and genres as input seeds.’
It is possible in the Spotify API to specify the track attributes which affect recommendations such as loudness, danceability, and valence. The researchers used four scenarios for the user task of selecting music. The used scenarios include: Rock night - my life needs passion; Dance party - dance till the world ends; A joyful after all exam and Cannot live without hip-hop.
Sweet spot
The researchers created eight experimental settings and conducted a between-subjects study, to explore the effect on cognitive load and recommendation acceptance for different personal characteristics. The participants with high musical sophistication perceived recommendations to be of higher quality, which in turn lead to higher recommendation acceptance. However, no effect of visual working memory on either cognitive load or recommendation acceptance was found. ‘This work contributes an understanding of how to design user control that hits the sweet spot between the perceived quality of recommendations and acceptable cognitive load.’
More information
Effects of personal characteristics on music recommender systems with different levels of controllability, Yucheng Jin (KU Leuven), Nava Tintarev (TU Delft) and Katrien Verbert (KU Leuven). Published in the RecSys '18 Proceedings of the 12th ACM Conference on Recommender Systems, pp 13-21, https://doi.org/10.1145/3240323.3240358
Homepage Nava Tintarev: http://www.wis.ewi.tudelft.nl/tintarev
Science Information Officer TU Delft Roy Meijer, +31 15 27811751, r.e.t.meijer@tudelft.nl