Behaviour in the energy transition
The energy transition requires behavioural and social change on a multi-actor level. Actors in the energy system like households, policymakers and organizations must adapt to huge technological, system and social change. Other actors like media, opinion leaders, lobby groups and political parties also interact in this complex system.
Understanding behavioural change and its precursors in such complex systems is challenging: the actors' underlying intra- and interpersonal psychological processes (e.g. bias in decision-making, preferences, roles, expectations, habits, group dynamics) vary widely and are difficult to identify.
Psychological theory provides insight into these processes. We believe that improving the scientific understanding of behavioural and social structures in the energy system will increase the effectiveness of tools that seek to support behavioural change.
One such tool is agent-based energy transition models. Agent-based modelling is a wonderful instrument to study the interaction between actors in the energy transition and is particularly useful when social constructs like reciprocity and feedback loops are suspected to exist, or when real world experiments are not feasible. To our knowledge, most existing agent-based energy transition models lack detail and profound scientific background when considering behavioural and social structures in the energy system.
In this research project we specifically aim to bridge the gap between behavioural science and agent-based system modelling. In order to do so, we translate psychological theory into re-usable building blocks for implementation in models, and we integrate behavioural and modelling research methods to improve data quality, thereby revolutionizing computational social science.
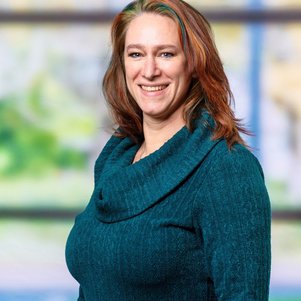