AI for Fluid Mechanics
The design and development of the next generation of energy efficient and cleaner aircrafts and wind farms will involve ever-more complex flows, thereby necessitating a deeper understanding of the physics involved and new analysis, prediction and control methodologies. Such advances can be obtained using recent developments in Artificial Intelligence and Machine Learning tools that have the potential to enable such breakthroughs.
Thanks to the capabilities of the aerodynamics group to generate large amount of data of physically relevant flows, using advanced experimental set-ups or high-fidelity simulations, we are able to fully leverage and exploit AI and ML-based algorithms while embedding physical knowledge within those data-driven methods. This provides the unique opportunity to deliver step-changes in our understanding and ability to predict and control complex flows.
The aim of our research in this field is in the development of such tools that combine recent advances in AI and our physical knowledge of fluid mechanics. This is applied to topics explored within the Aerodynamics group but also within the FPP department in general, and broadly covers:
- The control of laminar/turbulent boundary layer using AI and Reinforcement Learning
- The discovery of innovative aeroacoustic mitigation techniques
- The development of robust physics-based/data-driven closure models for CFD
- The surrogate modelling of turbulent flows with deep learning
- The identification of precursors to extreme events in transitional flows
Parts of these research activities are performed with the AI Fluid lab, a member of the Delft AI Labs network.
Current research projects
Control of Boundary Layer Instabilities with Deep Reinforcement Learning
The control of complex, transitional, and turbulent flows holds a core role within the general aerodynamic performance of modern transport and energy systems enabling the design of more efficient aircraft and wind farms. The development of a new category of AI-based active flow control systems able to autonomously control sensors and actuators to manage complex flows is the main motivation of this project. Intersecting the long-standing problem of boundary layer instability control with methodologies, tools, and ideas emerging from Artificial Intelligence is the primary innovation of the work. The main mechanism of transition control in this project is the suppression of the Tollmien-Schlichting waves (TS waves).
The TS waves play an important role in the process of laminar-turbulent transition on unswept wings in low freestream turbulence conditions. By suppressing the growth of TS waves in their early linear stage, it is possible to delay the transition process, extend laminar flow, and reduce skin-friction drag. Naturally occurring TS waves exhibit a nondeterministic spectrum of phases and frequencies which makes their control difficult when using traditional control loop techniques. In this research, advanced AI sensing and control algorithms like the Deep Reinforcement Learning algorithm will be developed to maximize both the effectiveness and robustness of engineering systems in which highly nonlinear flow behaviors and perturbations occur.
The development of such an AI-based control framework will be performed using data from numerical simulations and will be designed and demonstrated in such a numerical environment first. Subsequently, the implementation of the AI-based control will be implemented and tested in an experimental demonstrator to achieve sustainable and efficient transition delay on a developing TS-dominated boundary layer.
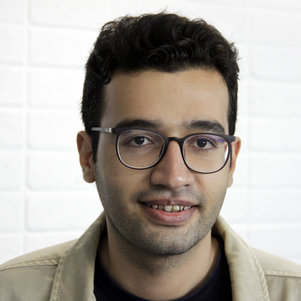
B (Babak) Mohammadikalakoo
PhD Candidate
✉ B.Mohammadikalakoo
@tudelft.nl
Data-driven characterisation and modelling of porous media inserts for the reduction of trailing edge noise
Increased human exposure to wind turbines resulting from the growing need for renewable energy production has led to a need for solutions aimed at reducing the noise generated by wind turbines. The use of porous media on the trailing edge of the turbine blades is promising in reducing turbulent boundary layer trailing edge noise.
The study consists in the creation of a data-driven approach aimed at identifying key porous material characteristics relevant to the noise reduction process in the context of porous inserts for the reduction of turbulent boundary layer trailing edge noise. This is performed in two steps: the relation between media topology and material characteristics must be established, and the material characteristics must be related to the noise reduction mechanisms. This deeper understanding of the interactions between porous media and grazing flows would then allow to tailor the porous media insert for a desired noise signature.
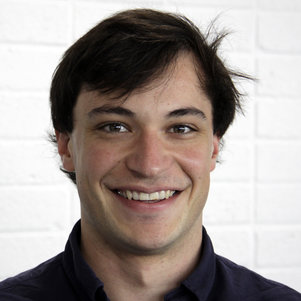
T.P. (Thomas) Hunter
PhD Candidate
✉ T.P.Hunter@tudelft.nl
Probabilistic surrogates for floating wind turbine load emulation
The broad scope of this study is to map the offshore environmental conditions to the fatigue and extreme loads on floating wind turbines using probabilistic surrogate models. Coupled aero-servo-hydro-elastic numerical simulations of such complex systems are expensive, warranting the need for faster load emulators. Data-driven approaches provide a flexible framework for surrogate model calibration. However, in the case of wind turbines, the response is significantly noisy due to random fluctuations in the inflow turbulence and irregular waves. As a result, the loads are random variables with an unknown probability distribution instead of deterministic values. The idea behind using probabilistic surrogates, like Bayesian and deep generative models, is to recover the complete conditional pdf of the response. The Ph.D. is a part of the STEP4WIND (https://step4wind.eu/) project, with TU Delft and Siemens Gamesa Renewable Energy.
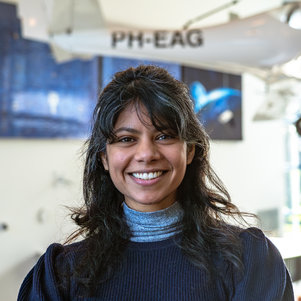
D. (Deepali) Singh
PhD Candidate
✉ D.Singh-1@tudelft.nl
Research Topic
Machine-learning of concise symbolic expressions for data-driven RANS closure modelling
Reynolds-averaged Navier-Stokes models are universally the model of choice for high-Reynolds number flow modelling in industry, being orders of magnitude cheaper than the scale-resolving alternatives. However, they suffer from significant modelling errors in many flows of interest. Data-driven modelling methods promise exploiting high-resolution experimental and DNS data to improve these models, using the techniques of machine-learning.
In this project we focus on techniques for deriving concise symbolic expressions for turbulence models, directly from data. The goal is to encode the dominant model corrections in the simplest possible way. By building simple corrections for several different classes of flows, we hope to move towards general-purpose data-driven turbulence closure models. Initial work will be focussed on designing a model for the NASA Collaborative Testing Challenge cases.
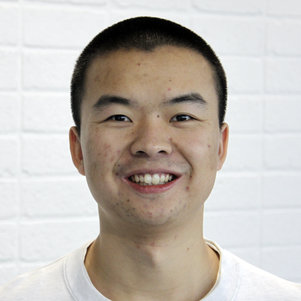
R. (Renzhi) Tian
PhD Candidate
✉ R.Tian-1@tudelft.nl
SpaRTA-based turbulence modelling for complex formula 1 flows
Tyler's research focuses on developing highly accurate turbulence models by integrating all forms of high-fidelity data with machine learning methods (SpaRTA) to produce accurate turbulence models for various complex flow problems originating from Formula 1 Racing. His project is part of the AI Fluids lab, with the goal to support Williams Grand Prix Engineering in improving their car's aerodynamic design through data-driven turbulence models.
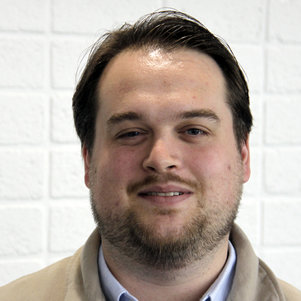
T.S.B. (Tyler) Buchanan
PhD Candidate
✉ T.S.B.Buchanan@tudelft.nl
Data-driven turbulence modelling for wind turbines
The drive to increase the size of wind turbines and wind farms requires the development of new methodologies able to model the increased complex physics such as the effects of atmospheric boundary layer, wakes and structural deflection. The aim of this project is to develop surrogate models using data-driven techniques to predict the aerodynamics loads based on numerical and experimental data.
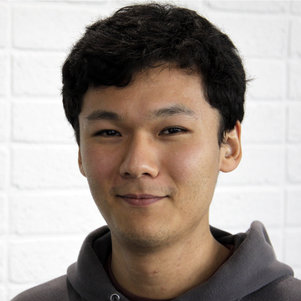
K. (Kherlen) Jigjid
PhD Candidate
✉ K.Jigjid@tudelft.nl
Reinforcement learning-based turbulence modelling for RANS
In this project, we aim to develop a deep reinforcement learning-based framework to improve turbulence models for Reynolds-Averaged Navier-Stokes simulations. This will consist in reframing the traditional turbulence modelling problem into a control problem where the machine learning-based agent is able to “correct” the flow through the turbulence model by adjusting the model or identifying a more suitable model structure. Such corrections will be based on enabling the RANS simulations to provide improved accuracy based on high fidelity flow profiles from experiments or simulations.
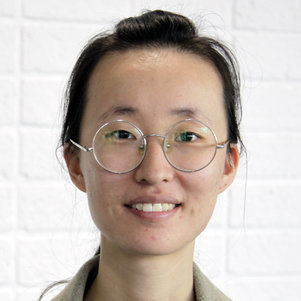
R. (Ruiying) Xu
PhD Candidate
✉ R.Xu-2@tudelft.nl
Fast modeling CO2 migration dynamics system with deep learning methods
Carbon capture and storage (CCS) is a viable technology to reduce the CO2 emissions and mitigate climate change. In CCS, the captured anthropogenic CO2 is injected into the geological media such as depleted reservoirs and deep saline aquifers for long-term sequestration. A deep-learning-based surrogate model is developed for predicting the temporal-spatial evolution of CO2 plume migration in heterogeneous geological formations with high computational efficiency, thus will be a significant predictive tool for engineers to manage the long-term process of CCS.
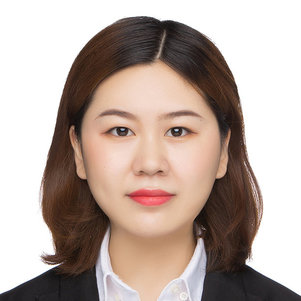
M. (Mengjie) Zhao
PhD Candidate
✉ M.Zhao-2@tudelft.nl