Coordinating Heat and Electricity Networks Operation and Planning using Data-Driven Approaches
Project description
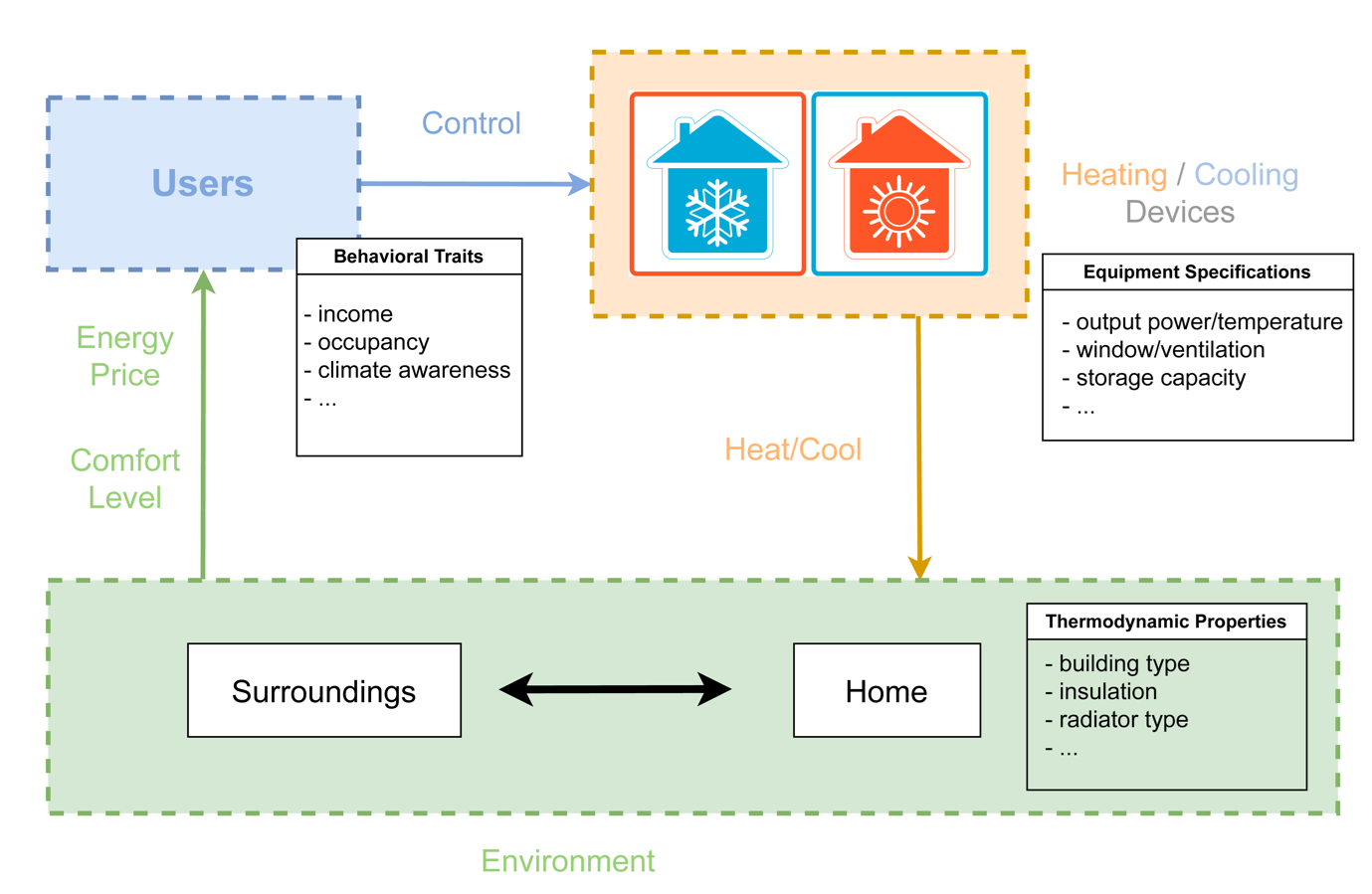
To meet the climate neutrality goals, the adoption of low or zero-carbon heat technologies must raise significantly and fast in recent future, heat pumps and district heating being the main choices. However, such technologies can have a major impact on the distribution network. This research aims to develop a coordinated optimal operation framework for future integrated electrical and heating systems by using data-driven models to conditionally generate high-resolution consumption profile data considering user behavior and building characteristics, quantifying heat demand flexibility with uncertainty, and employing optimization algorithms to find optimal operation plans. This goal will be achieved by answering the following research questions:
• Research question 1: How to leverage data-driven methods to generate heat consumption profiles for time series analysis based on aggregated conditions?
• Research question 2: How to use data-driven models to predict future heat consumption profiles with uncertainty bounds based on behavioral, building, and system level information?
• Research question 3: How to quantify the potential heat flexibility of a neighborhood based on behavioral and building information?
• Research question 4: How to coordinate future heat and electricity operation considering heat flexibility to maximize utilization of current infrastructure?
The above research questions are vital but challenging. First, the heat consumption data is high-dimensional and has complex mathematical structures. Balancing the expressivity and computational complexity of the models is a difficult task. On top of that, integrating cross-sector information, namely behavioral and building information, into the data generation require advanced models combining data-driven and engineering approaches. Modelling flexibility is another challenge. Defining flexibility in a sensible way and modelling the time-varying nature are both intricate tasks. Lastly, the coordination of a future electricity and heat network using the generated data and quantified flexibility requires a new and rigorous optimization framework.
Despite the challenges, there are clear and promising approaches to deal with them. High-dimensional data modelling can be done with the new state-of-the-art deep generative models, diffusion models. Such models show both diversity and fidelity in the generated data. They also inherit the scalability of previous deep learning models, computationally efficient to model high-dimensional data. These models are also good at taking in cross-sector information and can accept incomplete input conditions with the help of attention mechanism. Besides, the copulas, a conventional machine learning method, is being considered as an alternative. As for the demand flexibility, I am aiming at adopting the flexibility function approach, which models the user demand from a control system’s perspective. With all these models established, the optimal coordinated operation can be solved in an mixed-integer optimization framework and assessed by a new security assessment framework.
Intended deliverables
• Three to four journal papers from the research results of the four research questions
• Two to three conference papers

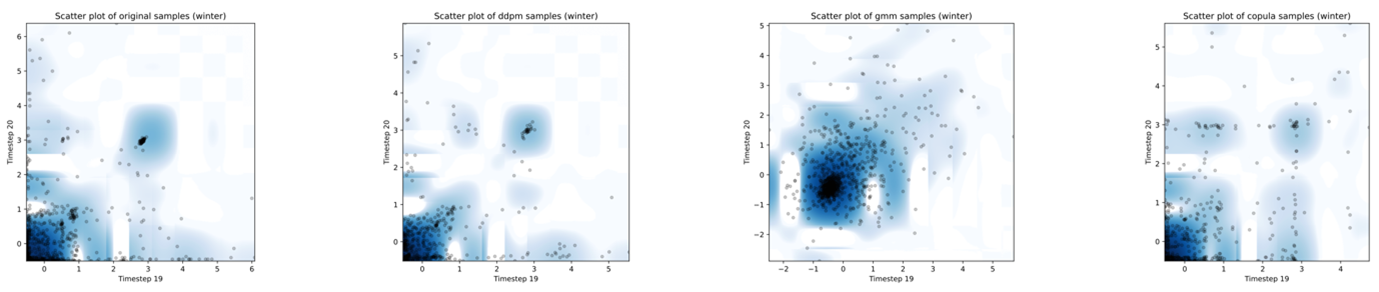
Project team
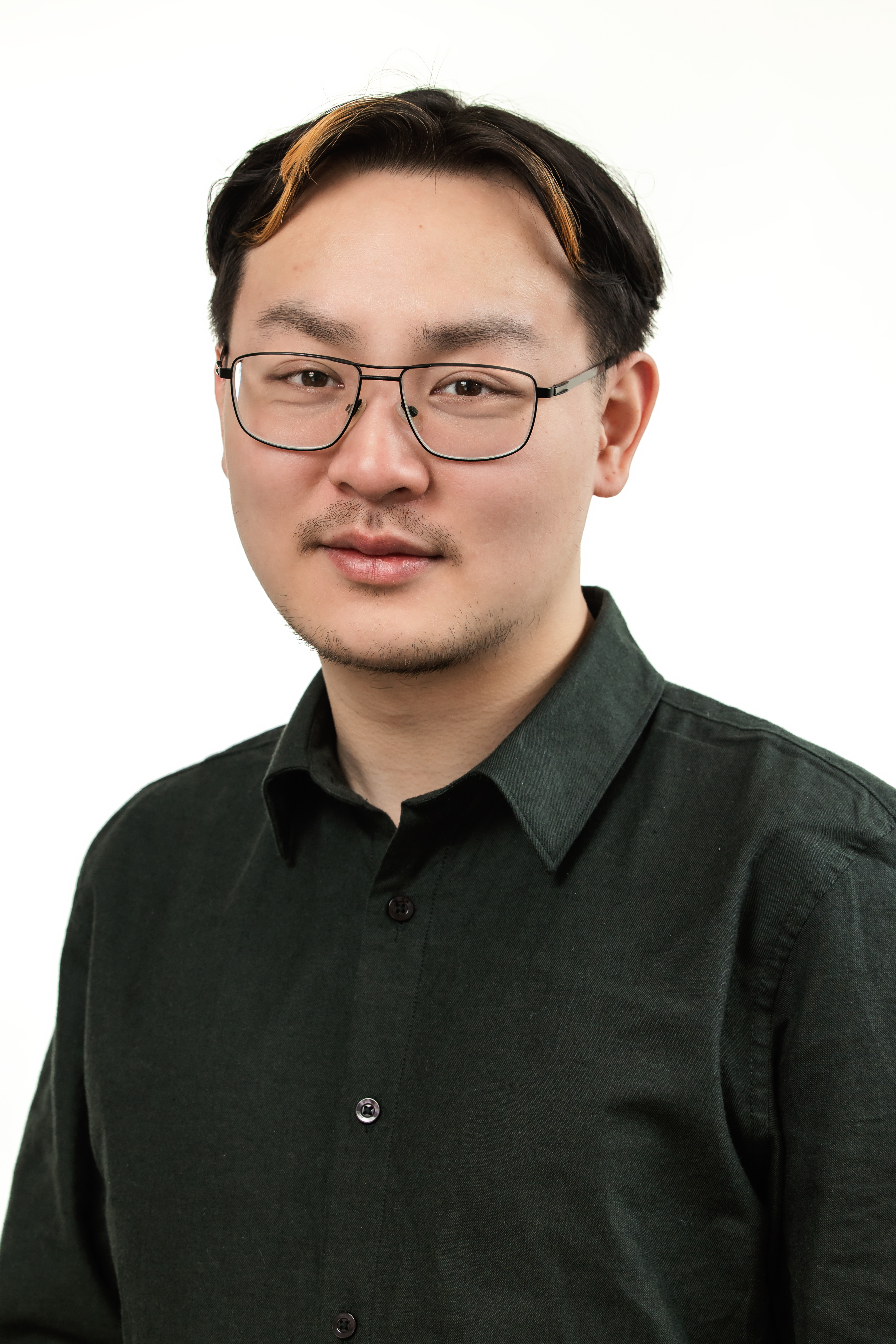
PhD Researcher:
N. (Nan) Lin
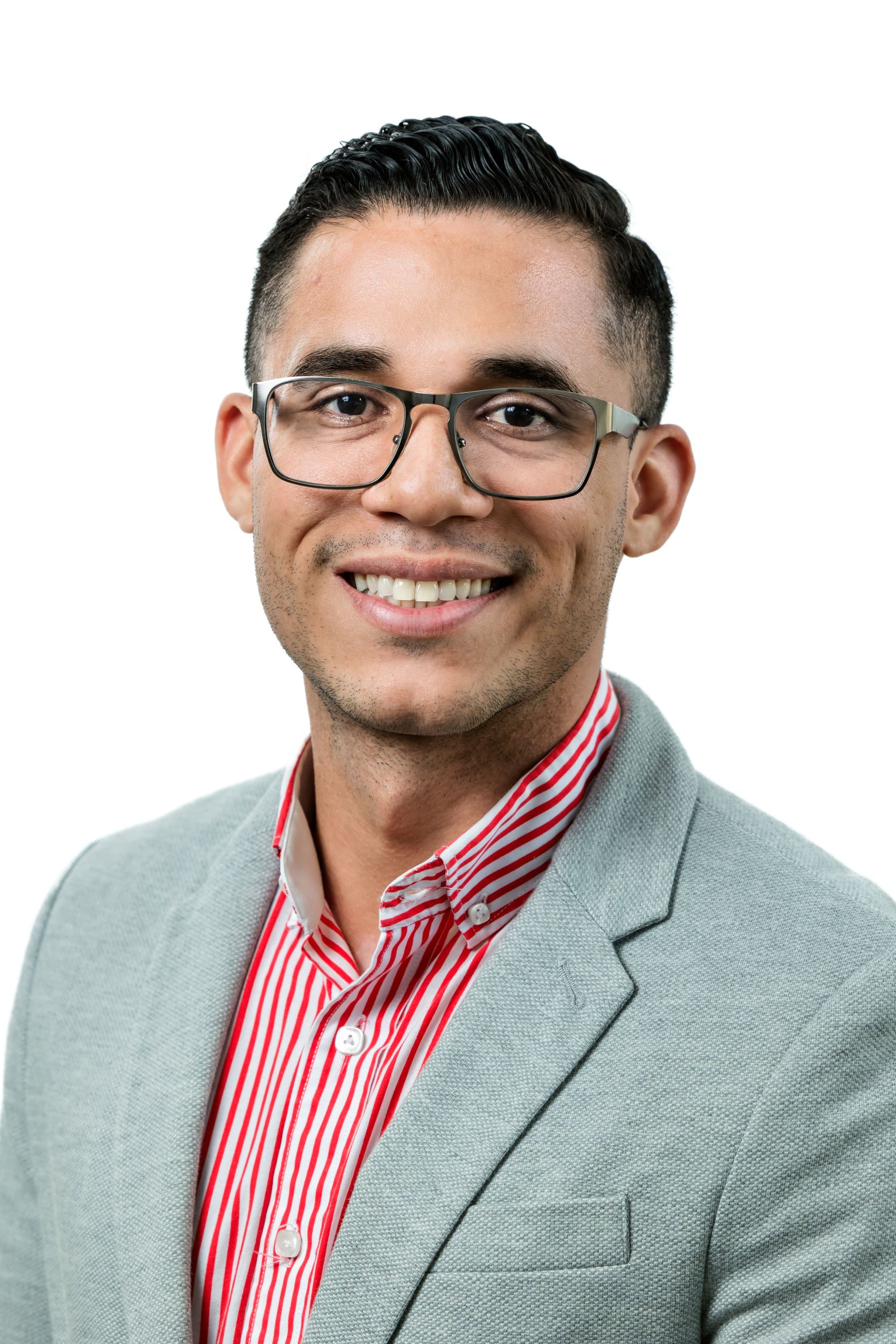
Co-promotor and Daily Supervisor:
P. (Pedro) P. Vergara Barrios
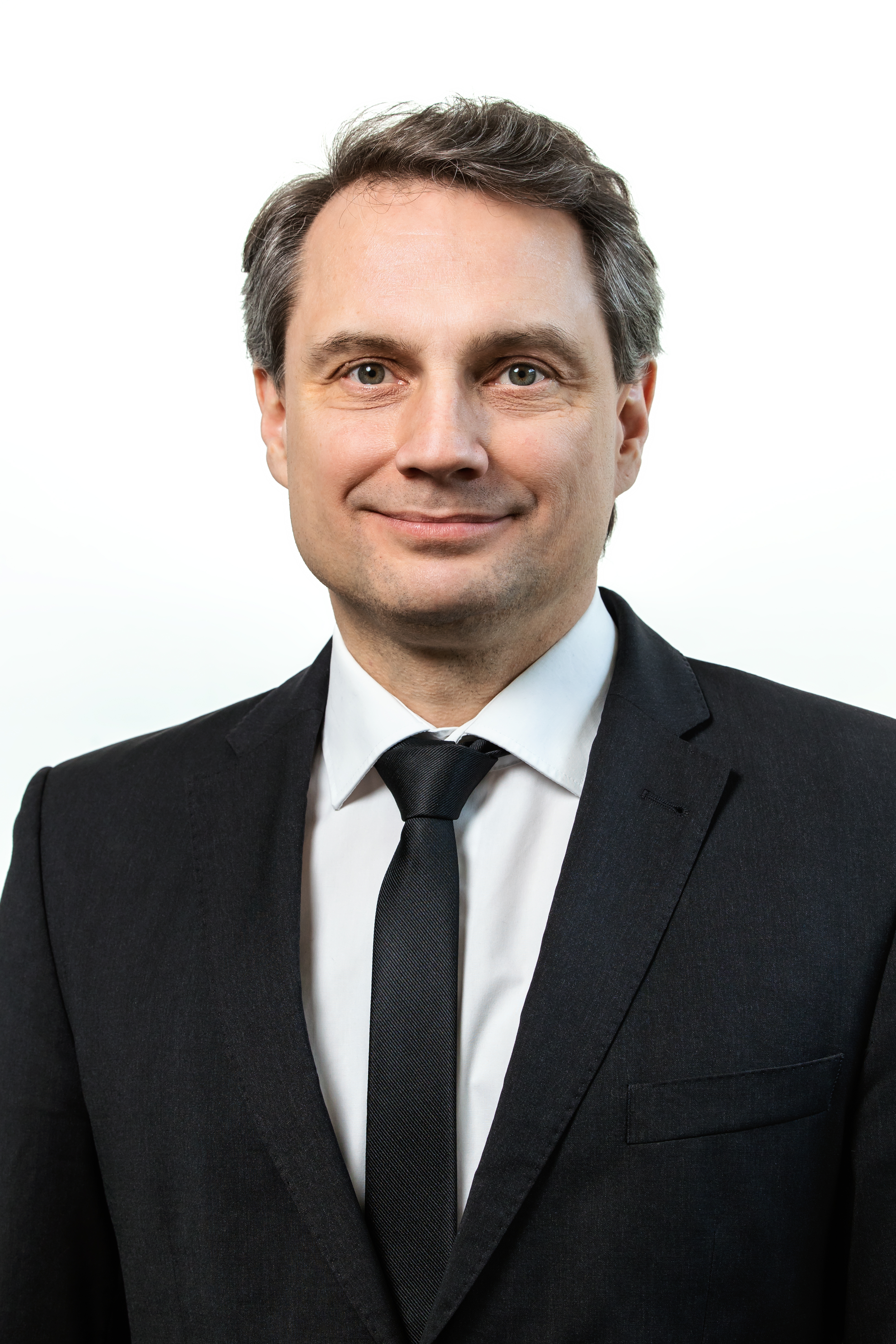
Promotor:
Prof.dr. P. (Peter) Palensky
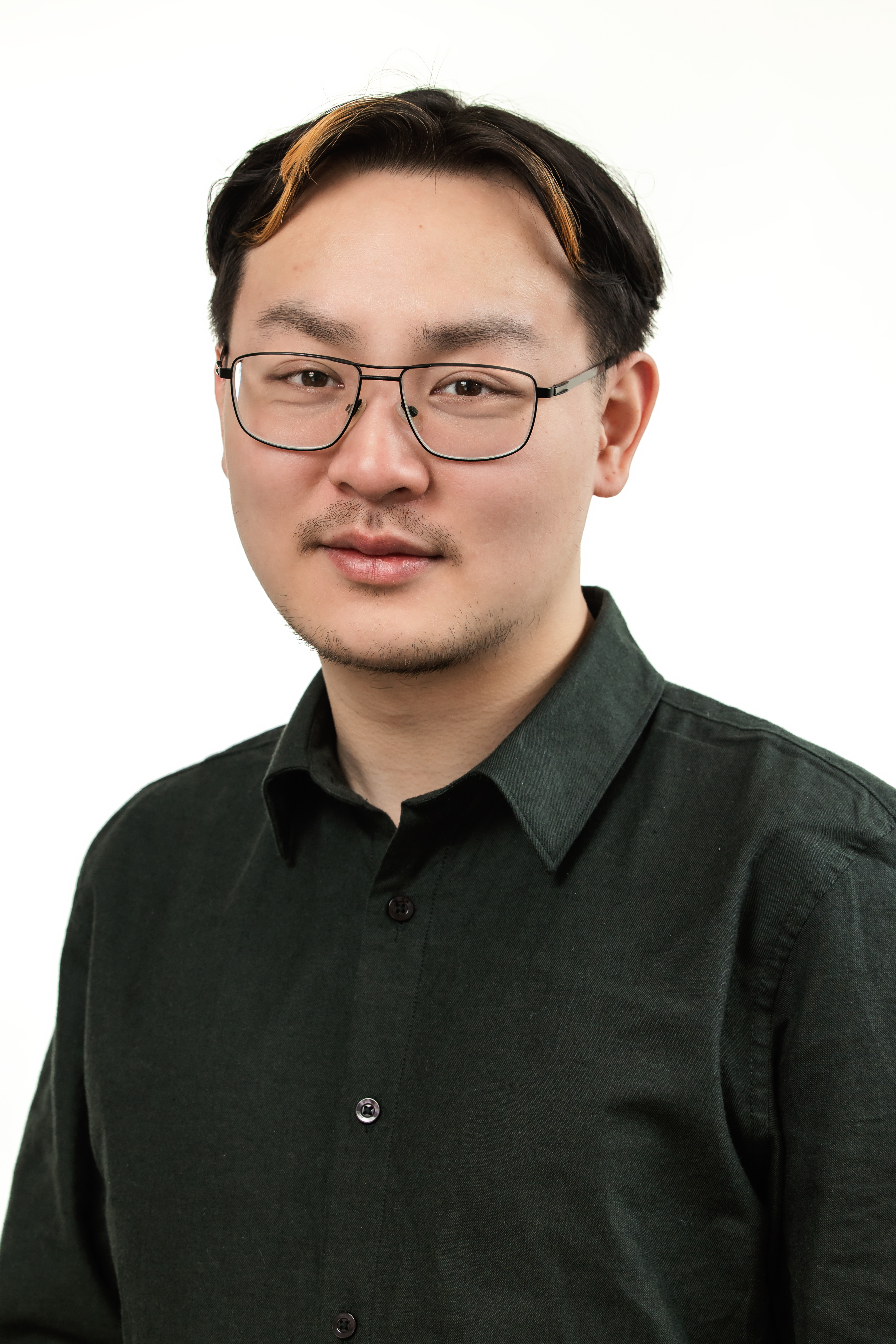
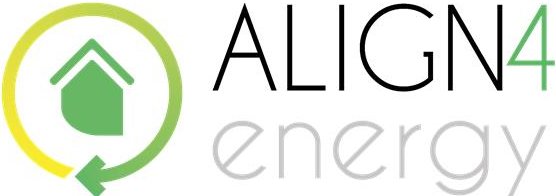
N. (Nan) Lin
Aug. 2022, I received my M. Sc. Degree in Electrical Engineering also from TU Delft, in the Circuits and Systems Group under the supervision of dr.ir. Justin Dauwels and dr. Hadi Jamali-Rad. My research interests are in machine learning or deep learning from a statistical or probabilistic perspective. More specifically, I am interested in Bayesian learning, copula models, deep generative models, transfer learning, and reinforcement learning. Currently, I am focusing on applications in power systems as my primary job. I also keep myself updated on graph machine learning and computer vision as a side interest.