Guillermo Ortiz Jiménez
Faculty of Electrical Engineering, Mathematics & Computer Science
Guillermo obtained his master’s degree in Electrical Engineering. He chose a graduation project in the field of signal processing.
He first dived into the topic of deep learning, a machine learning technique that teaches computers to learn by example. He examined whether he could extend classical deep learning techniques, meant to classify audio signals or images, to techniques useful for classifying signals supported by an irregular structure. Such learning methods can be used to classify complicated non-structured data such as measurements from body sensors. Guillermo managed to quickly develop a graph-convolutional deep network structure, which successfully classified the irregular signals.
Guillermo also tackled the topic of sparse sensing, a technique to reduce the number of sensors in a sensing system. He established a complete sparse sensing framework. His work can, for example, help movie recommender systems (such as Netflix) to pick movies and users in a clever way to predict all preferences. Guillermo is the first to introduce such a framework and his work is truly ground-breaking. A conference paper has already been accepted for one of the main conferences of the IEEE Signal Processing Society. His thesis was rewarded with the highest possible grade (10).
“With Guillermo you can always have very mature discussions and brainstorming sessions with interesting outcomes. He is one of the best MSc students I ever met.”
Graduation committee - Prof. GJ.T. Leus, Dr S.P. Chepuri, Dr R. Hendriks, Dr D. Tax
Thesis synposis
In this new era of data science, Machine Learning (ML) and Signal Processing (SP) are becoming the key driving forces of the fourth industrial revolution. They are paving the way for the genesis of new disruptive applications across many fields, ranging from the biomedical sciences to the ICT and manufacturing industries. Most of the tools in ML and SP, however, can only be applied to signals residing on a regular grid, e.g. audio and image signals. In my thesis, hence, I extended these tools to signals that lie on irregular domains with a graph structure, e.g. traffic networks, 3D meshes, or social network graphs. In particular, I developed two mathematical frameworks: one for the classification of graph signals using tools from deep learning and one for their sampling. The applications of my thesis are diverse varying from brain signal decoding (classification) to the automatic recommendation of items in e-commerce (sampling).
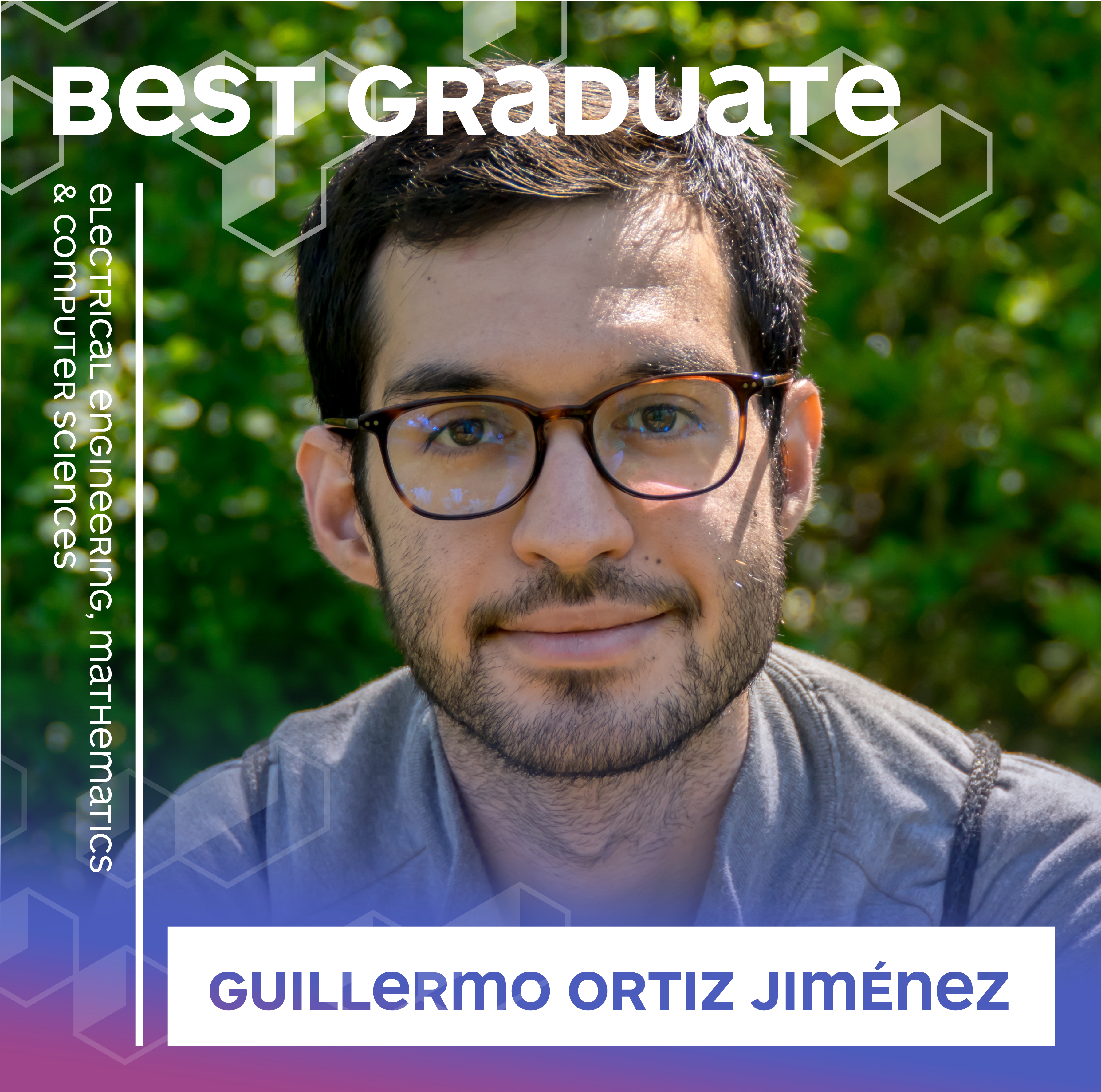
