There is no shortage of methods that use data to assess and predict resilience. Resilience tools, driven by data and AI, increasingly influence human decision making in planning and crisis management. While there is as such a wide range of dashboards and models, it is unclear what the implications are of the methodological choices and assumptions underlying the assessments. This lack of understanding of what the implications are of using different tools may lead to biased decisions.
This project will build on the foundations set in previous projects of the lab to assess urban and social resilience, and investigate the implications of using different methods (PCA, SOM), parameter configurations, or data sets to determine resilience. This will allow us to study the implications of methodological choices under different scenarios, especially for rapid change and crises. In a second step, you will empirically explore the explainability of the models and model results. How can phenomena, that are inherently complex, be explained and understood via a resilience model?
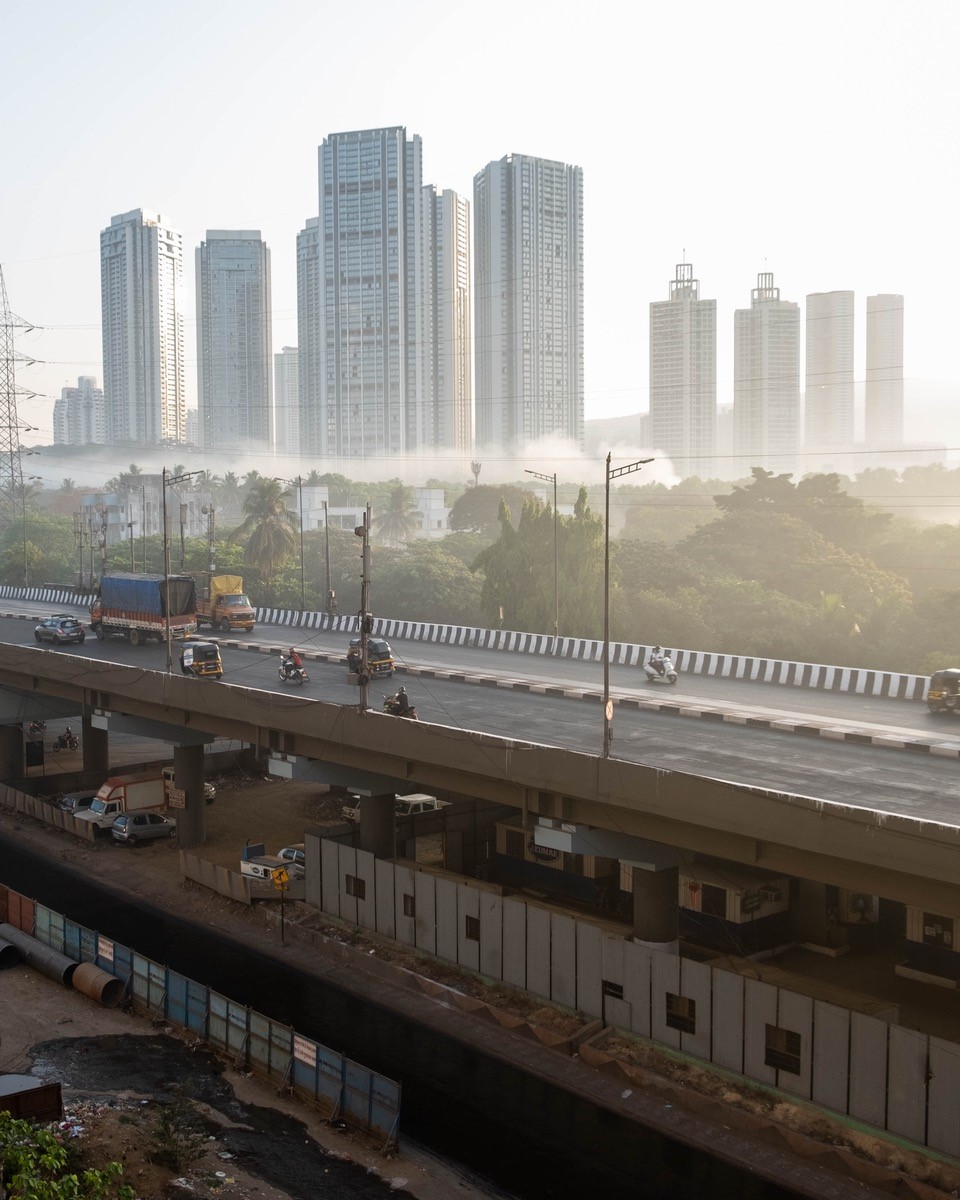