Project Summary
In the race towards building smarter, more powerful and more connected machines, scientists are also searching for ways to make sure they are energy efficient. If no action is taken, computers will soon need more electricity than the world energy resources can generate. More alarmingly, the Internet of Things will connect up to 50 billion devices to the cloud through wireless networks. This EU-funded APROPOS project is exploring ways to decrease energy consumption and optimise energy-accuracy trade-offs. To that end, it is developing approximate computing, in which applications using data may be satisfied with an 'acceptable' level of accuracy, and it will train early-stage researchers in energy-accuracy trade-offs on circuit, architecture, software and system-level solutions.
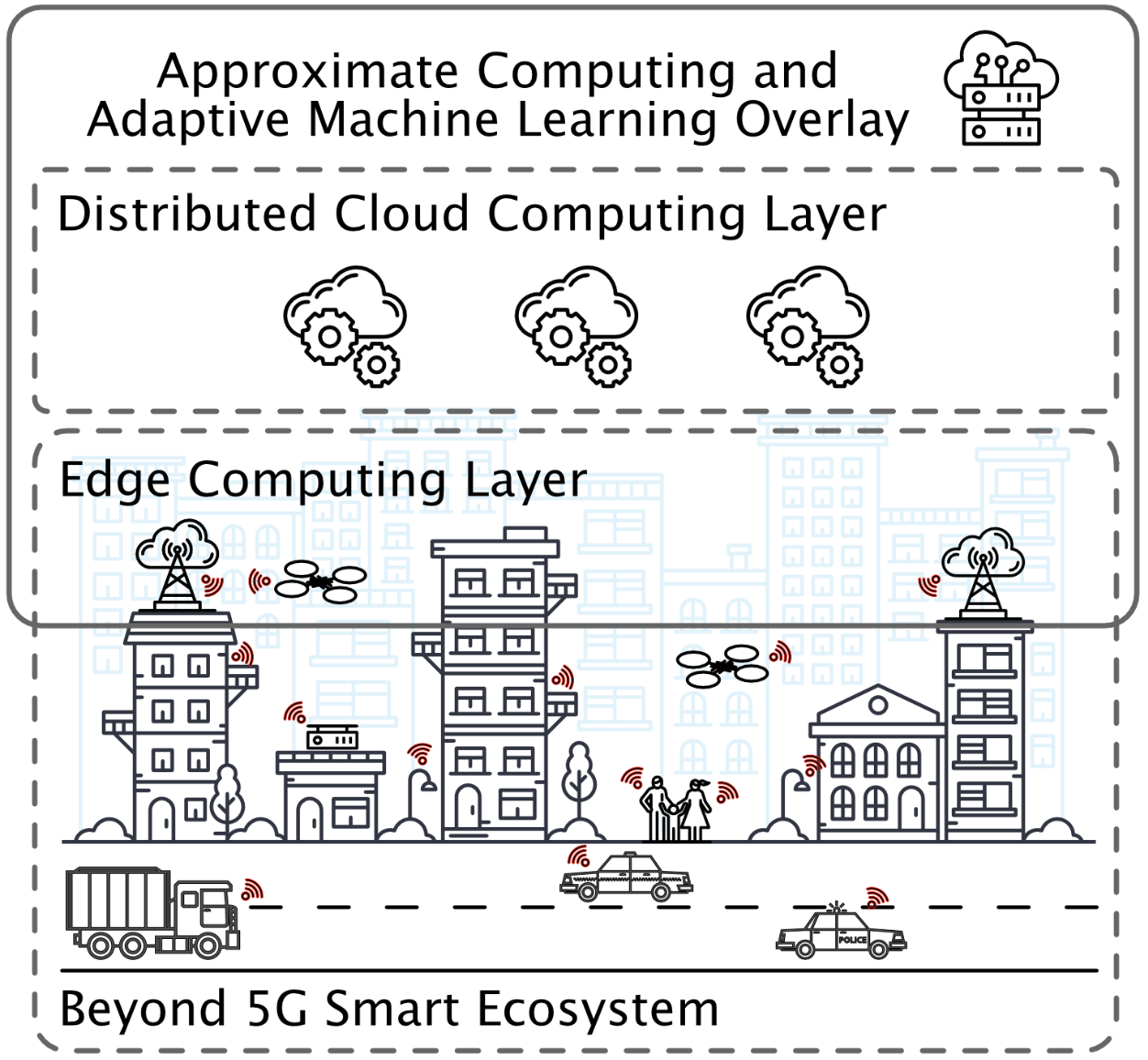
Project description
APROPOS project has received funding circa €4M from the European Union’s Horizon 2020 (H2020) Marie Sklodowska-Curie Innovative Training Networks H2020-MSCA-ITN-2020 call, under the Grant Agreement no 956090. The doctoral network of APROPOS will train 15 PhDs to tackle the challenges of future embedded and high-performance computing energy efficiency by using disruptive methodologies.
The APROPOS PI at TU Delft is Dr. Aaron Ding, whose team is investigating disruptive methodologies to tackle the challenges of future AI and embedded computing energy efficiency.
Following the current trend, by 2040 computers may need more electricity than the world energy resources can generate. To make things worse, Internet-of-Things will soon connect up to 50 billion devices through wireless networks to the cloud. APROPOS aims at decreasing energy consumption in both distributed computing and communications for cloud-based cyber-physical systems.
In APROPOS, we propose adaptive Approximate Computing to optimize energy-accuracy trade-offs. Luckily, in many parts of the global data acquisition, transfer, computation, and storage systems there exists the possibility to trade off accuracy to less power and less time consumed. As examples, numerous sensors are measuring noisy or inexact inputs; the algorithms processing the acquired signals can be stochastic; the applications using the data may be satisfied with an “acceptable” accuracy instead of exact and absolutely correct results; the system may be resilient against occasional errors; and a coarse classification may be enough for a data mining system. By introducing a new dimension, accuracy, to the design optimization, the energy efficiency can even be improved by a factor of 10x-50x. We will train the spearheads of the future generation to cope with the technologies, methodologies, and tools for successfully applying Approximate Computing to power and energy saving. The training, in this first ever ITN addressing approximate computing, is to a large extent done by researching energy- accuracy trade-offs on circuit, architecture, software, and system-level solutions, bringing together world leading experts from European organizations to train the ESR fellows.
What's Next?
Despite the recent advances in semiconductor technology and energy-aware system design, the overall energy consumption of computing and communication systems is rapidly growing. On one hand, the pervasiveness of intercnonected systems has drastically increased the demand for computing and communications. On the other hand, the user expectations on features and battery life of on-line devices are increasing all the time, creating another incentive for finding good trade-offs between performance and energy consumption. To improve the energy efficiency of computing and communications, radical efforts are needed. We postulate that, by allowing a decrease in the accuracy of processing at different parts of the computing chain, we can achieve less power consumption and shorter computing time. Trading off accuracy for improved power consumption and/or performance is generally known as Approximate Computing (AC), which is he central focus of this EU Marie Curie ITN project.