Building a sustainable future using Digital Twins
What do urban planners dream about? It might look a lot like a city-building game: to fly above a city, adding solar panels or installing double-paned glass everywhere, and instantly see the effects on energy use. Then maybe implement localised renewable energy, plant some trees, and replace all the sidewalks. Now, how resilient is your neighbourhood to a changing climate? This dream is becoming reality in the dizzying world of Digital Twins. And these already powerful tools are becoming even better and more accessible thanks to current developments in AI.
Note: AI was used in the creation of the text and images of this article
How to build a Digital Twin
Digital Twins are virtual replicas which amalgamate a plethora of data sources to create an accurate virtual representation of an environment. The complexities of real-world implementations have long constrained attempts to increase cities’ sustainability, energy-efficiency, and resilience. But Urban Digital Twins could serve as dynamic simulations that help assess the impact of interventions. Dr. Azarakhsh Rafiee and her colleagues at the Digital Technologies group aim to use these tools to aid in the energy transition. Their main datasets are:
In addition to all these sources, changes over time must be incorporated. These changes include the aging of buildings, periodic maintenance, and the addition of new structures. When done right, the Digital Twin becomes a virtual laboratory to simulate a myriad of interventions—ranging from enhanced insulation to solar panel installations and localized electricity grids.
The real power of AI emerges in extracting useful information from all these data sources. Machine learning algorithms establish intricate relationships between variables, producing a holistic model that mirrors the studied neighbourhood more efficiently than could be achieved previously. And besides its ability to create accurate models in less time, AI is pivotal in the Digital Twin framework for several other reasons:
- Satellite Imagery Analysis: AI's object recognition capabilities expedite the creation of highly accurate maps from satellite imagery, further reducing time and resource investments.
- Enhanced Predictive Modelling: AI's ability to determine trends based on historical and real-time sensor data constantly refines the Digital Twin's accuracy in iterative predictions of the future.
- Enhanced Scenario Modelling: AI's modelling power significantly enhances the ability to incorporate future developments such as trends in material availability or a shifting climate, offering stakeholders improved insight for a more oriented scenario design.
AI can improve Urban Digital Twins by finetuning their ability to forecast the future
The effective integration of AI in Urban Digital Twins holds far-reaching implications. With the Digital Twin acting as a predictive testing ground, urban planners can make informed decisions to:
- Boost Energy Efficiency: By simulating energy-efficient upgrades, such as improved insulation and optimized solar panel placements, planners can gauge potential energy savings and environmental impacts.
- Enhance Resilience: The Digital Twin facilitates resilience assessment against environmental factors like heatwaves or storms. This enables planners to design structures and neighbourhoods that can withstand future challenges.
- Optimize Urban Layout: AI-driven modelling aid in designing urban spaces that promote sustainable living, reducing energy consumption and fostering a higher quality of life.
- Plan Infrastructure Development: From the design of localized renewable energy resources to efficient waste management systems, AI-integrated Digital Twins assist in identifying the most effective infrastructure strategies.
In addition to these advantages, Urban Digital Twins could stimulate community engagement. The transparent visualization provided by the Digital Twin offers citizens a comprehensible platform to understand and influence urban development decisions.
Digital Twins with enhanced AI could enable us to better prepare for environmental dangers
In the evolving realm of urban planning, the fusion of AI, Digital Twins, and Geographic Information Systems paves the way for groundbreaking advancements. Urban Digital Twins have emerged as a tool of unparalleled power, enabling planners to predict, experiment, and optimize interventions in urban landscapes with remarkable precision. As AI technologies continue to advance, the prospect of sustainable, energy-efficient, and resilient cities becomes more attainable than ever before. The intricate dance between data, algorithms, and urban visionaries is shaping a future where our cities evolve intelligently, benefiting both present and future generations.
What is LiDAR?
LiDAR (Light Detection And Ranging) technology gathers data by emitting rapid laser pulses from an airborne or ground-based sensor. These pulses hit surfaces and objects, then bounce back to the sensor. By precisely measuring the time it takes for these pulses to return, LIDAR calculates the distance to each point. Collecting millions of distance measurements creates a detailed point cloud. This ‘cloud’ is processed to generate a three-dimensional map, accurately capturing the topography and structures of, for example, a neighbourhood.
In 1996 a project was set up called AHN or ‘Actueel Hoogtebestand Nederland’ (Current Height Data Netherlands). In the course of seven years, AHN created a height map of the entire country by using LIDAR systems mounted on planes and helicopters. And a few years later they repeated the entire process. Currently, the project is on its way to deliver the fourth version of their map, with increased accuracy and resolution. You can freely access the data by going to their website.
Published: September 2023
More information
- GIS Technology is one of two Chairs of Digital Technology Section within AE&T. Their focus is the application of big data in decision-making, from the level of buildings to international regions.
- Azarakhsh Rafiee is part of the DATALESs (DATA-driven Localised Energy Systems) project. Specifically, she is involved with ‘Digital Twins for decentralized local energy system design’, which studies Dutch neighbourhoods and will run until 2026.
Header image: Generated by the tools of Geodan and it is their exclusive property
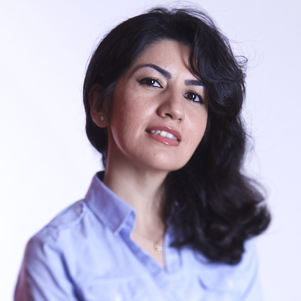