Sicco Verwer
Sicco Verwer is an Associate Professor in machine learning with applications in cyber security and software engineering at TU Delft since 2014. Before this, he has been a postdoctoral researcher for several years at RU Nijmegen, KU Leuven, and TU Eindhoven.
He has worked on several topics in machine learning and is best known for his work in grammatical inference, i.e., learning state machines from trace data. He has researched and implemented several algorithms for learning such models including RTI, which is one of the first that is able to learn timed automata. In 2013, he received a VENI grant from STW to extend this work and apply it in cyber security. Other recent work include several methods for declarative modelling of machine learning problems using mathematical solvers, and making classifiers discrimination-aware.
He teaches two courses in the cyber security master at TU Delft: Cyber Data Analytics and Automated Software Testing and Reverse Engineering.
If you are interested in the research performed by his lab, or joining as PhD or MSc student, please have a look at Sicco's publications and past publicly available MSc and BSc theses.
Er zijn geen resultaten gevonden, of Pure is tijdelijk onbereikbaar.
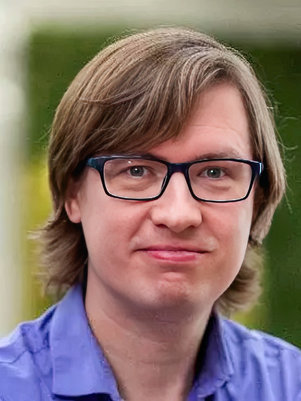
Dr. S.E. Verwer
- s.e.verwer@tudelft.nl
-
EEMCS, Algorithmics
P.O. Box 5031, 2600 GA Delft
The Netherlands