Sicco Verwer
Sicco Verwer is an Associate Professor in machine learning with applications in cyber security and software engineering at TU Delft since 2014. Before this, he has been a postdoctoral researcher for several years at RU Nijmegen, KU Leuven, and TU Eindhoven.
He has worked on several topics in machine learning and is best known for his work in grammatical inference, i.e., learning state machines from trace data. He has researched and implemented several algorithms for learning such models including RTI, which is one of the first that is able to learn timed automata. In 2013, he received a VENI grant from STW to extend this work and apply it in cyber security. Other recent work include several methods for declarative modelling of machine learning problems using mathematical solvers, and making classifiers discrimination-aware.
He teaches two courses in the cyber security master at TU Delft: Cyber Data Analytics and Automated Software Testing and Reverse Engineering.
If you are interested in the research performed by his lab, or joining as PhD or MSc student, please have a look at Sicco's publications and past publicly available MSc and BSc theses.
- Christian Hammerschmidt, Benjamin Loos, Radu State, Thomas Engel, Sicco Verwer, Flexible State-Merging for learning (P)DFAs in Python, In Proceedings of The 13th International Conference on Grammatical Inference Volume 57 p.154-159, JMLR.
- Nino Pellegrino, Christian Hammerschmidt, Sicco Verwer, Qin Lin, Learning Deterministic Finite Automata from Innite Alphabets, In Proceedings of The 13th International Conference on Grammatical Inference Volume 57 p.69-72, JMLR.
- Yihuan Zhang, Qin Lin, Jun Wang, Sicco Verwer, John M. Dolan, Lane-Change Intention Estimation for Car-Following Control in Autonomous Driving, In IEEE Transactions on Intelligent Vehicles Volume 3 p.276-286.
- Qin Lin, Sicco Verwer, John Dolan, (2020), Safety Verification of a Data-driven Adaptive Cruise Controller
- Sicco Verwer, Christian A. Hammerschmidt, flexfringe: A Passive Automaton Learning Package, In 2017 IEEE International Conference on Software Maintenance and Evolution, ICSME 2017 p.638-642, IEEE.
- Christian A. Hammerschmidt, Sebastian Garcia, Sicco Verwer, Radu State, Reliable Machine Learning for Networking: Key Issues and Approaches, In 2017 IEEE 42nd conference on Local Computer Networks, LCN 2017 p.167-170, IEEE.
- Jason. S. Rhuggenaath, Yingqian Zhang, Alp Akcay, Uzay Kaymak, Sicco Verwer, Learning fuzzy decision trees using integer programming, In 2018 IEEE International Conference on Fuzzy Systems (FUZZ-IEEE) p.1-8, IEEE.
- Daniël Vos, Sicco Verwer, (2023), Adversarially Robust Decision Tree Relabeling, In Machine Learning and Knowledge Discovery in Databases - European Conference, ECML PKDD 2022, Proceedings p.203-218, Springer.
- Rowan Hoogervorst, Yingqian Zhang, Gamze Tillem, Zekeriya Erkin, Sicco Verwer, (2019), Solving bin-packing problems under privacy preservation: Possibilities and trade-offs, In Information Sciences Volume 500 p.203-216.
- Yingqian Zhang, Sicco Verwer, Qing Chuan Ye, Auction optimization using regression trees and linear models as integer programs, In Artificial Intelligence Volume 244 p.368-395.
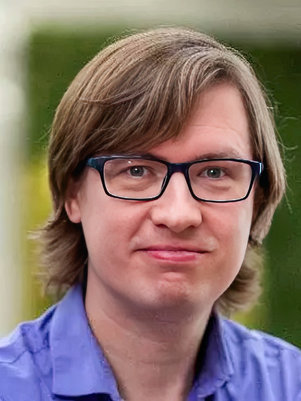
Dr. S.E. Verwer
- s.e.verwer@tudelft.nl
-
EEMCS, Algorithmics
P.O. Box 5031, 2600 GA Delft
The Netherlands