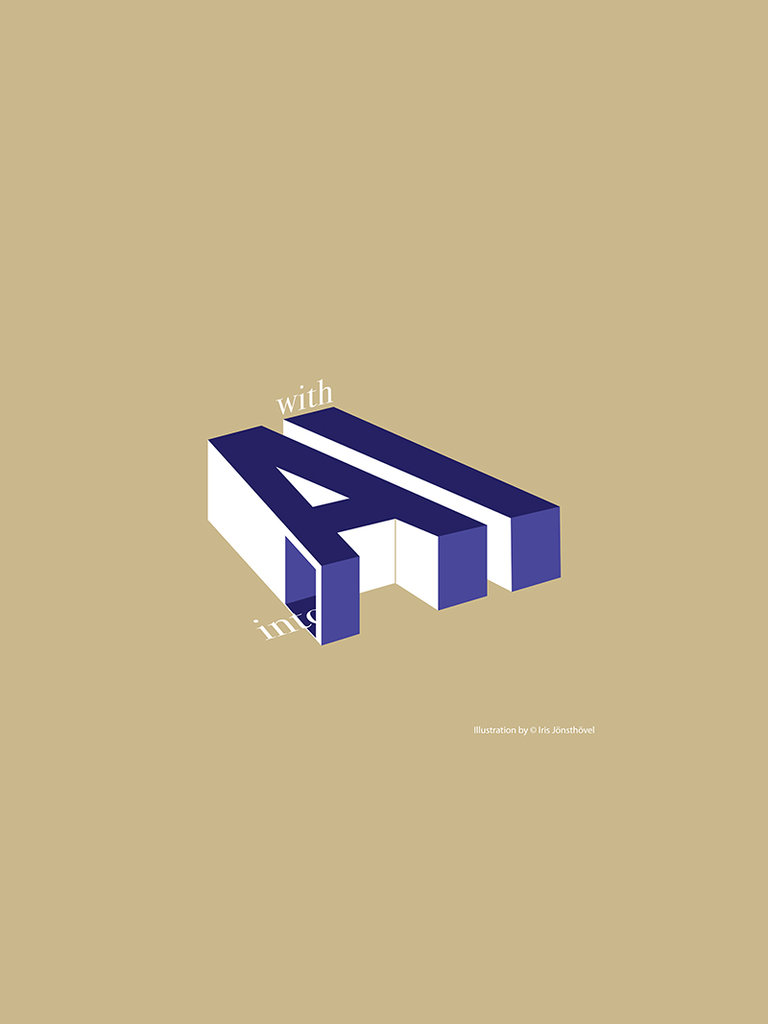
Looking ahead for smart logistics
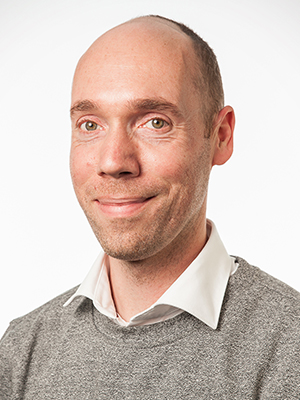
Rudy Negenborn

Matthijs Spaan
TU Delft’s AI research is offering the port and transport sector solutions for growing transport needs. Researchers Prof. Rudy Negenborn and Dr Matthijs Spaan explain why collaboration is important in this process.
TU Delft’s AI research in the port and shipping sector is developing smart computer systems that make smart logistics possible. This will enable us to deploy the infrastructure and available equipment more cheaply, sustainably and efficiently. It is a key area of TU Delft’s research and also part of AI Hub Zuid Holland's 'AI ecosystem’, in which the university is collaborating with the universities in Rotterdam and Leiden and the business community. “Applied AI is not just about technology," explains Prof. Rudy Negenborn, professor of multi-machine operations & logistics. “You also need to take account of economics, law and ethics. Each university has its own specific focus. In the AI hub, we bring everything together."
Different decision-makers
Negenborn summarises his field of research as “smart shipping and smart logistics”. Rather than being available in a single place, information about port transport is spread across different automation systems and organisations. “That means that a decision on something like how fast a ship should travel to a certain destination with a certain cargo is not made by a single computer or person, but by different decision-makers." His research focuses on the scale and spread of decision-making in logistics. Negenborn is developing algorithms that apply data from computer systems and sensors in the field to optimise the transportation of goods across water, road and railway networks and is focusing on algorithms to improve maritime transport by means of autonomous ships.
Reducing risks
Matthijs Spaan, associate professor in computer science, is also working on algorithms. He is exploring how they can make decisions based on predictions, in order to anticipate what might happen, both operationally (in the next hours) and in the long-term (years). This could prove useful for a port-based chemicals factory, for example, that aims to optimise energy consumption. Spaan: “With data concerning the current situation alone, the system is unable to anticipate. In that case, it can only learn through trial and error. But that can be dangerous, or damaging to the system itself." Systems that are capable of anticipating can reduce risks. Spaan: “The great thing is that knowledge from this fundamental research can also be applied outside the maritime domain."
Collaboration between AI and humans is an important theme. Transport operators still tend to interact a lot, via telephone or email. Negenborn: “If you use smart AI systems to share more information more rapidly, you can coordinate better and make decisions more efficiently. For example, when deciding which ship should land with which cargo and when."
Clash of interests
Human-system interaction is also important in guaranteeing human control of AI. Imagine that variations in wind speed cause multiple ships to sail too closely together. This causes a clash of different interests: safety, efficiency, cost-effectiveness. Can an AI system make decisions about that? Spaan is incorporating these kinds of questions in the models. “We teach systems when a prediction is certain enough for the systems to decide for themselves and when they have to suggest several scenarios for a human to decide from."
Collaboration also helps to tackle the challenges faced in using AI applications in maritime transport: the limited availability of real-life data to enable algorithms to learn. Shipping companies and port terminal operators are competitors and can be reluctant to share data. But this does not deter the researchers. Negenborn "We’re collaborating with companies. If necessary, we create databases from real data, but omit any sensitive company information. “
Large network
The fact that companies still lack awareness of the potential and limitations of smart systems is another challenge. But thanks to TU Delft’s large network, students can do graduation projects at companies. This enables them to experience the kind of problems where AI can prove helpful. At the same time, the teaching about AI is not limited to the AI disciplines alone. For example, students of mechanical engineering also learn about the possibilities AI offers. Spaan: “This means that students from different disciplines spend time at lots of different companies. That strengthens collaboration and enhances corporate appreciation of AI."