Artificial Intelligence in Fluid Mechanics
AI and fluid mechanics: for better planes and wind farms
Designing more efficient aircraft and wind farms, requires an ever deeper understanding of continuously more complex flows as we strive towards a carbon neutral society.
With the advent of new experimental techniques and high-fidelity flow simulations that provides big data on complex flows, AI tools can now be leveraged to provide this better understanding and prediction capability for those complex flows. In the AIFluids Lab, we focus on two major challenges of fluid mechanics: predicting and controlling complex, unstable and turbulent flows by using new AI techniques combined with past physical understanding of flows. We aim to combine human and machine insights to get to the essence of complex physical flows, be it in air, water or other media.
Using this combination of AI and physics-based approaches, our research will lead to models that can be used to design more efficient aircraft and wind farms. It will also allow us to train our AI algorithms so that they can autonomously control sensors and actuators to manage complex flows and improve aerodynamic performance.
The AIFluids Lab is part of the TU Delft AI Labs programme.
The team
Directors
Courses
2024/2025
2023/2024
2022/2023
2021/2022
-
Test, Analysis & Simulation | AE2223-I
2020/2021
-
Test, Analysis & Simulation | AE2223-I
2019/2020
-
Test, Analysis & Simulation | AE2223-I
Resources
For MSc thesis projects at the AIFluids lab, see the “MSc AE Profile Aerodynamics” page on Brightpage or take contact with our lab by sending an email.
Master projects
Ongoing
- Data-driven turbulence modelling for magneto hydrodynamics, Anh Khoa Doan, Alejandro Montoya (2023/2024)
- Knowledge-based, deep learning and hybrid reduced order modelling of chaotic systems, Anh Khoa Doan, Jochem Veerman (2022/2023)
- AI-based identification of large scale structures in turbulence, Anh Khoa Doan, Austin Ramanna (2022/2023)
- Neural Networks for Aerodynamic Modelling, Anh Khoa Doan, Vincent Maes (2022/2023)
- Data-driven techniques for flashback detection and suppression in reheat hydrogen combustion, Anh Khoa Doan, Mihnea Floris (2022/2023)
- Velocity reconstruction of turbulent pool fires using physics-informed neural networks, Anh Khoa Doan, Pablo Gonzalez (2022/2023)
- Delay of laminar-to-turbulent transition via reinforcement learning, Anh Khoa Doan, Babak Mohammadikalakoo (2021/2022)
- AI-driven design of porous surfaces for noise reduction, Anh Khoa Doan, Thomas Hunter (2021/2022)
- AI-driven nonlinear turbulence models, Davide Modesti, Ruiying Xu (2021/2022)
- AI-driven turbulence models for flows in wind farms, Davide Modesti, Kherlen Jigjid (2021/2022)
Finished
- Machine learning-based identification of precursors of extreme events in chaotic, Anh Khoa Doan, Urszula Golyscka (2022/2023)
- Data-Driven URANS Closure with Sparse Regression, Anh Khoa Doan, Nicolo Miori (2022/2023)
- Physical Reconstruction in Pool Fire with Deep Learning, Anh Khoa Doan, Dirk de Boer (2022/2023)
- Generalizing Simulation Surrogates with Physical Information and Transfer Learning, Anh Khoa Doan, Tadeusz Kaniewski (2022/2023)
- Turbulent flows learning with deep learning, Anh Khoa Doan, Rohan Kaushik (2022/2023)
- Physics-informed neural networks for highly compressible flows, Anh Khoa Doan, Thomas Wagenaar (2022/2023)
- Physics-Informed Neural Networks for Fluid Mechanics, Anh Khoa Doan, Dobbin Huang (2021/2022)
Partners
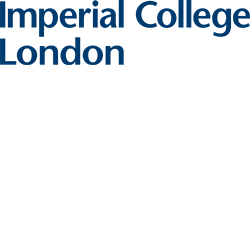
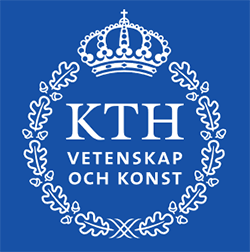
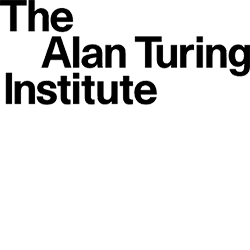
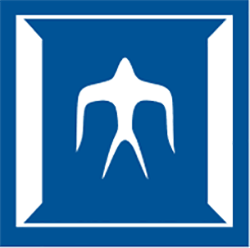
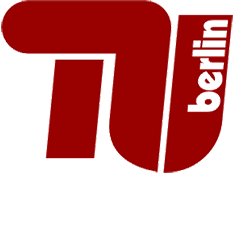
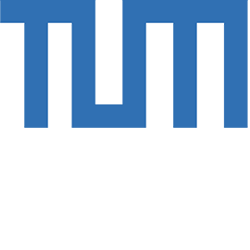